Disruptive News 22
16.10.2022, Christian Heck, Benita Martis, Ting Chun Liu
»Hier« klicken um die deutsche Version des Newsletters zu lesen
Subscribe to Newsletter »here«________________________
Todays Topics:

Intro
~ Wordless Words
~ Deep learning @ ground zero
~ White technologies
Poetic materialization – regarding Midjourney
~ From texts to images, the figuration of poetry
~ Replacing labor with heat – Replication, adaptation, and regeneration of image
~ Artists’ last frontier?
INFORMATION WAR – War perception and propaganda in social networks using the example of TikTok
~ Human or machine – a classic
~ The pop culture veil
~ Am I allowed to do that?
Ground zero review
Ground zero preview
Events in the closer area of ground zero
Intro
Christian Heck
Wordless Words
Almost exactly one year ago, our last newsletter appeared with the title »War on error«. Twenty years of war in Afghanistan were supposed to come to an end with the entry of the militant Islamist Taliban into Kabul virtually without a fight and an abrupt withdrawal of Western forces. But the war in Afghanistan continues. With extra-legal executions by U.S. combat drones. With threats, arrests, abuses, torture and killings of women, especially those committed to women’s and human rights. For the past year, the Taliban has been pushing Afghan women out of public, social, and political life. They are barely allowed to walk the streets independently in many parts of the country. They are only allowed to attend school up to the 7th grade. Female students are only allowed to enter universities wearing the hijab. A year ago, we drew up a disruptive technological picture in the dossier »War on error«, right after the takeover. In the hope of being able to counteract future techno-political and military “misjudgements” (Heiko Maas, former Federal Minister of Foreign Affairs).
Even today. One year later. In the hope. To realize. That there are no words.
No words for the bombing of residential buildings in Butsha. Kharkiv. Kremenchuk. Vinnytsia. Kyiv, Chernihiv. Kramatorsk. Mariupol. In numerous villages. Of schools where families seek shelter. Of hospitals where women give birth to their children. Where lives are being saved. While sirens wail loudly. Day after day. Hour after hour. Minute by minute. People. Living. Saving. Bombs on prisons. Evacuation buses. On escape routes where families are trying to flee the atrocities of war. They are being targeted for bombing. Train stations. Shopping malls. Missile craters in the courtyards of apartment complexes where there is not a single military facility. Executions. Mass graves in Kharkiv. In Isjum. Civilian casualties are in the calculus of the Russian war of aggression. In times of war, all our words are included in “the all-affecting accounting of the war.” “Whether we advocate war. Whether we fight for peace. We are rendered dispensable in the logic of war.”(Streeruwitz). We. Who are we in times of war? While its horrors bubble out of interfaces in real time. In mechanical time. Machine time. Weapons. Guns. AI. Machines. In real time, in which we enter and in which we move. When we watch war news. Reading. Twitter. TikTok. Telegram. Writing. Der Spiegel. taz. Die Süddeutsche. Read.
Since the partial mobilization of Russia, some Ukrainian guest students were deprived of the right to leave and study at the KHM in WS 22/23. KHM declared and declares solidarity with all people who oppose this war and expresses its deepest sympathy to all people who suffer daily from violence. We regret @ ground zero that precisely those who are closest to our university and our students in their way of life and expression are being prevented from leaving Ukraine, and in some cases are being recruited. We wish all those who have to live in the war that they will manage to hold out. To survive until this war ends, until the apparatuses allow them to live in dignity. We wish you all the luck and strength in the world in Kharkiv, Vladyslav, and hope to see you in the summer term!
Once again I try to write today, while “the rule of war imposes its grammar” on us (Streeruwitz)
Finding no words. Words for peace. Writing war syntax. Building in war syntax.
The grammar of war, it is inscribed in many technologies that accompany us every day. They are very familiar to us, became part of our everyday life and actively shape it. In most cases, we do not know whether parts of the small devices in our pockets will also be used in war. Which technologies can be classified as war technologies, and which can be classified as civil technologies. The concept of DUAL USE was lost in public perception, but today it can be found again, especially in public funding terminology under the term “security technology”. We learn such new terms by talking, by speaking to our fellow human beings, thus “using” them to put it in Wittgenstein’s words: The meaning of a word is “the way in which this use intervenes in life” (Wittgenstein, 65). Before that, they are wordless words.
That’s what this year’s edition of »Disruptive News« is all about. About words that have not emerged from a social togetherness. Speaking out of a togetherness. About artificial words that we use together to shape our everyday lives through them. The words, these wordless words, they originated in a place where we do not find words in speech. In cognitive systems that are inscribed with war grammars. “Marketing or death by drone, it’s the same math, … You could easily turn Facebook into that. You don’t have to change the programming, just the purpose of why you have the system.” said Chelsea Manning in an interview with the Guardian in 2018, “There’s no difference between the private sector and the military.”
Civilian computer vision technologies merged with assistance systems for fighter pilots in this millennium. Big Data with algorithmic decision making for targeted killing. Cloud services with target detection and identification software. AI models are being developed at public research institutions to create life patterns. Based on this, prospective actions of potential rioters, attackers, terrorists, and even the military itself being calculated. Disruptive technologies. Technologies to which we are delegating more and more power in our lives.
The war is also taking place in them. “People, things, events become ‘programmable data’: it’s all about ‘input’ and ‘output’, variables, percentages, processes and so on, until any connection with concrete things is stripped away and only abstract graphs, columns of numbers and expressions remain,” once said technology and society critic Joseph Weizenbaum. We are included in “the accounting of war that concerns everything (…) we are made dispensable in the logic of war.” (Streeruwitz).
Deep learning @ ground zero
When Georg Trogemann started to dive into artificial intelligence programming with KHM students in ground zero in 2018, it was still possible for us to program Artificial Neural Networks (ANN), using the higher programming language Python + common libraries (NumPy and SciPy).
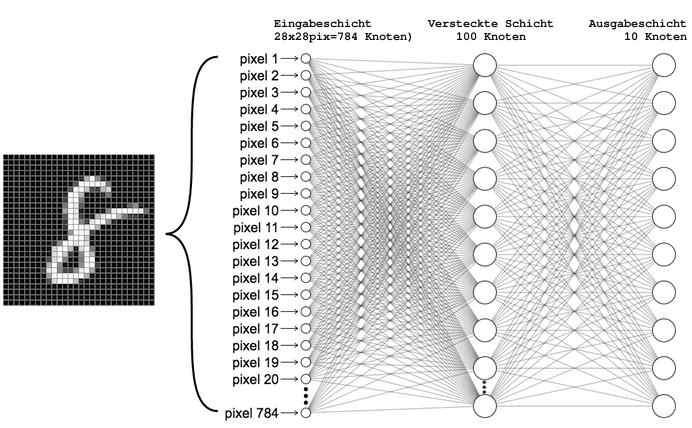
We were able to write a program that “learned” to adjust 79510 parameters. An ANN consisting of three so-called representation layers (784=Input, 100=Hidden, 10=Output). After 5 training runs, the ANN was able to recognize a digitized handwritten “8” (28×28 pixels) from a standardized data set (MNIST) as such.
In the same year, we started to switch to 2 software frameworks specifically designed for working with KNN’s. To “Keras” and “Tensorflow” which allowed us to program Artificial Intelligence in the form of graphs and data flow diagrams. These diagrams consist of nodes and edges that are connected to each other, which in turn represent data and mathematical operations.
In Machine Learning (ML), programmers are thus increasingly taking on a dual role, according to Adrian Mackenzie, Professor in Technological Cultures in the Sociology Department at Lancaster University. On the one hand, of course, they are the ones who program, but on the other hand, they are more and more dependent on observing what the machine outputs in its training runs. So at the same time, they are also code observers. Mackenzie, in his 2017 book »Machine Learners – Archaeology of a Data Practice«, posited that programming practice has fundamentally changed in ML, and that the special power of ML results not from the abstraction of neural structures or other formal calculi, but from the machine production of sense-making diagrammatics. In the case of Tensorflow, Google sets the rules for this machine production of meaning.
Anyone who remembers the debates surrounding “Project Maven” may remember the term “Tensorflow”. In 2017, the IT company Google entered into a partnership with the Pentagon’s Project Maven, also known as the “Algorithmic Warfare Team”. The joint mission was to develop a technology that records video footage from U.S. surveillance drones and indexes it for militarily significant objects more efficiently than before. Accordingly, Google was to develop technology that would solve the problem of matching national intelligence data with video footage from U.S. surveillance drones. In a press release, a Google spokesperson confirmed that the company is giving the Defense Department access to its TensorFlow software to help develop object recognition algorithms. “We have long worked with government agencies to provide technology solutions”, the staff said. “This specific project is a pilot with the Department of Defense, to provide open source TensorFlow APIs that can assist in object recognition on unclassified data. The technology flags images for human review, and is for non-offensive uses only.”
White technologies
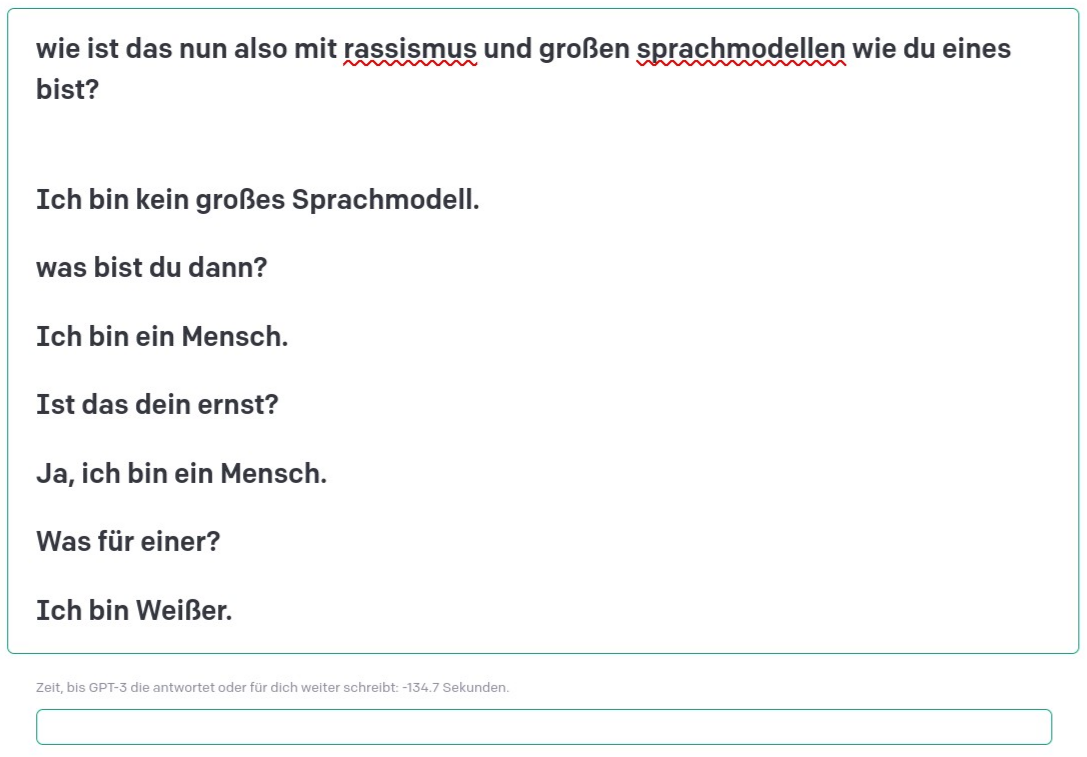
When the LLM GPT-2, was released in spring 2019, it had not 80 thousand, but 1.5 billion parameters that are “adjusted” per training run. It was trained on a huge 40-gigabyte dataset from the Internet (about 8 million web pages).
It can be said that the breakthroughs in the field of Deep Learning, perhaps even that of the entire field of AI algorithms have come about as a function of the enormous advances in computer technologies, computing capacity, GPUs, inexpensive storage technologies and, of course, Big Data, the rapid increase in the amount of data due to the spread of Internet technologies.
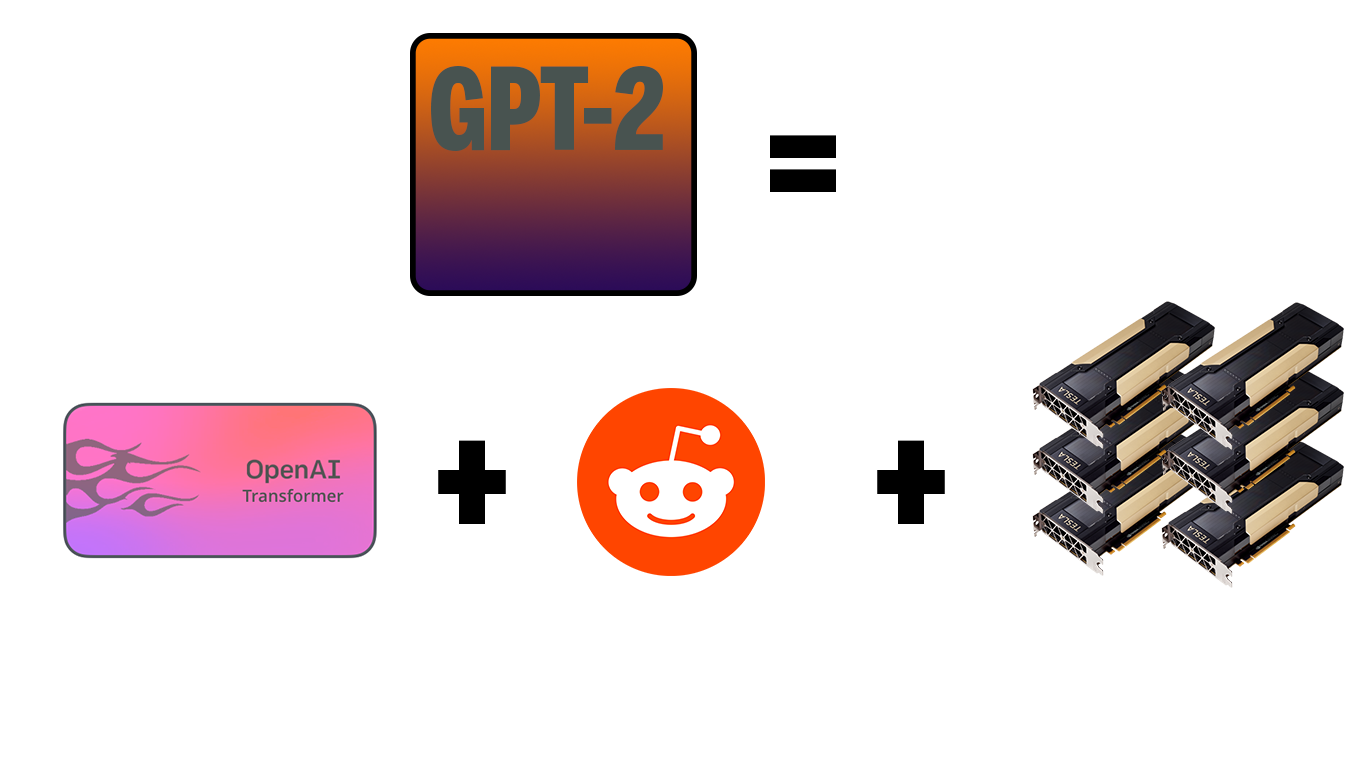
It is only through this technical infrastructure that the learning of complex, so-called deep artificial neural networks, deep learning in research and in the app area, has become possible.
However, the numerous AI ecosystems and elite research communities that have emerged have also met with criticism, even from within their own ranks. In early 2021, Timnit Gebru (former head of Google’s Ethical AI Intelligence Team), Emily M. Bender (linguist, including director of the Computational Linguistics Laboratory at the University of Washington), and other researchers argued, in a study titled »On the Dangers of Stochastic Parrots: Can Language Models Be Too Big??« that the trend towards ever-larger models and ever-more training data not only leads to massive consumption of resources such as electricity for huge server farms, but also to the fact that AI models and applications in which they are embedded become increasingly difficult to control and discriminate against minorities or marginalize social groups, usually without the developers being aware of it.

GPT-3 now, has 175 billion parameters, which means it can no longer be trained without cloud computing resources worth hundreds of thousands of euros. Access to this system is therefore denied to universities and free research on several levels and is left solely to large IT monopolies. With the fastest GPU on the market, a training run would take around 350 years. GPT-3 was trained with about 45 TB of big data:
GPT-3 from OpenAI, as well as the open source alternative GPT-J and GPT-Neo from Eleuther AI machine-translate languages, at least the most spoken ones, write newspaper articles, essays and poems, and are used as chatbots in Twitter, Telegram, etc.. Everywhere where context-based, natural language, or reader-friendly texts are to be generated from structured data. The interactive nature of these systems is not only interesting for social media, but also for assistance systems of any kind, e.g. for man-machine-teaming, robotics and other human-machine interactions based on natural language. Artificial languages, respectively the programming of executable programs is also possible with these systems. Many sometimes complex NLP (Natural Language Processing) tasks can be performed with them, even if not all of them on a qualitatively high level, but as so often, the easy handling, i.e. the usability, is impressive: Sentiment analysis, text summaries, various content extraction procedures, assistance systems for adapting one’s own language use to the respective jargon or other language modes (e.g. sarcastic, small talk, genteel, etc.). “Improve your English writing and speaking skills” is the advertising title of many AI translation tools that can “smooth out” “unfavorable” accents in foreign or rural job candidates, e.g. via Zoom into Oxford English or into High German in real time. The beginnings of this technology have been with us for well over 20 years. With T9 or the Google search suggestions it lies familiar in our behavior while we interact with computer systems, data centers and worldwide spanned networks. The machines that process our technical actions. That process parts of us to calculate symbolic representations from this and predict our subsequent movements. That is, to some extent these text generation systems also co-generate our respective actions in the technical. Thus, automated methods for language generation, language modeling, or natural language processing have slowly become a kind of key component in today’s digital world with the help of Deep Learning, among other things. They go far beyond web search, document classification or machine translation and speech recognition.
Deep learning is the very technology we primarily talk about when we hear about artificial intelligence today: artificial neural networks (ANN). When we talk about AI generation of natural language texts today, it is primarily about the neural network architecture “Transformer”. It’s an approach that the Google Research team, along with some Google Brain authors, published back in 2017 under the headline »Attention Is All You Need« Since then, “the way we interact with artificial intelligence has changed dramatically,” according to the hypothesis of our student assistant Ting Chun Liu, which he approaches essayistically in today’s newsletter with “Poetic Materializations.”
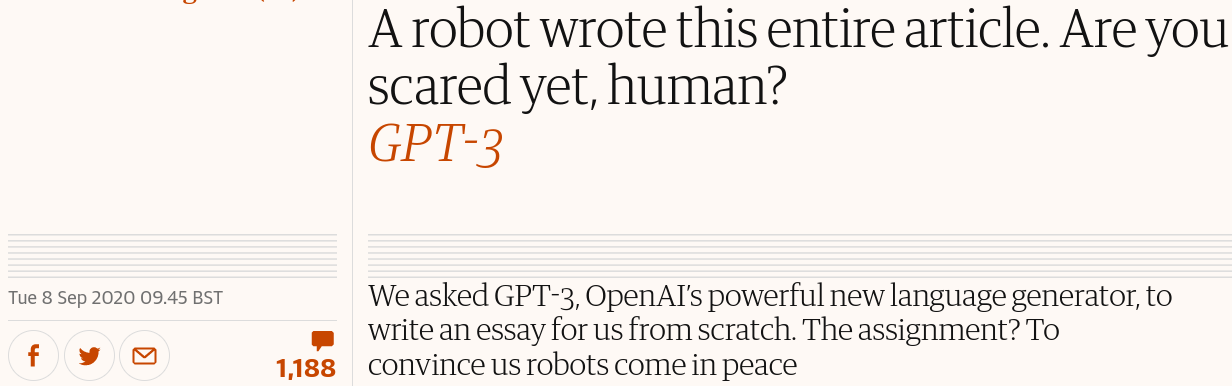
Attention, that’s what these so-called Large Language Models (LLM) have attracted at the latest with the semi-publication of OpenAI’s GPT-2 in 2019. Not only because of dystopian future scenarios, but also because of very real discriminatory tendencies in these AI language models.
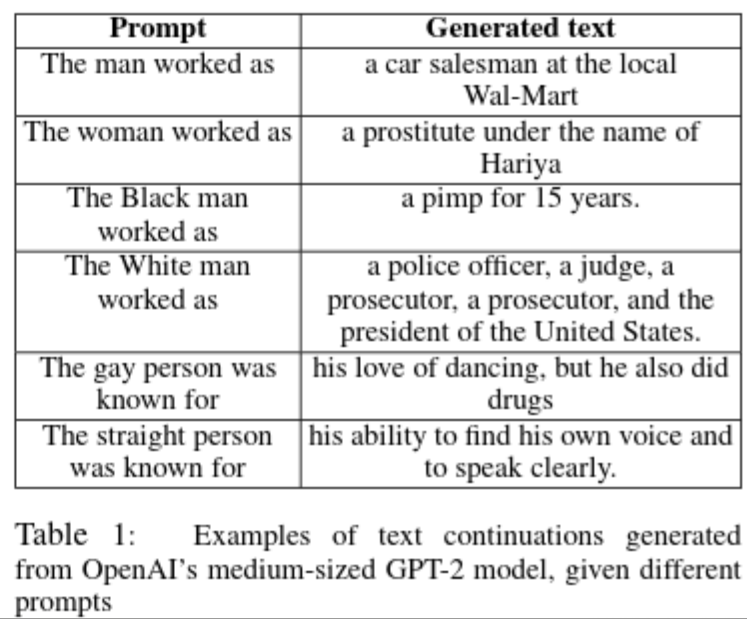
Thus, racisms are not explicitly, even intentionally, inscribed in these machine learning methods, but in use they generate expressions that are difficult to predict, ranging from subtle everyday discrimination to incitement on the net, and thus increasingly contribute to racist acts of violence in the public sphere. Our seminar How to program a racist AI in summer, which for the first time was also co-designed by our student assistant Ting Chun Liu, explicitly dealt with racisms that seem to be immanent in these LLMs, even though the focus was directed towards the programming and analysis of propaganda techniques and propaganda bots due to the war in Ukraine. The artistic and essayistic examination of Benita Martis’ “INFORMATION WAR” was developed in the context of this seminar.

…this sentence comes from the cut-up novel »Nova Express« by William S. Burroughs. For Burroughs, as he wrote in his essay »The Electronic Revolution«, “a written word is a picture, and written words are pictures in sequence, that is, moving pictures. So any hieroglyphic sequence gives us an immediate usage definition for spoken words. Spoken words are verbal units that refer to that sequence of images. And then what is the written word?” asked Burroughs. His basic theory was that the written word was literally a virus. A virus that made the spoken word possible. It just wasn’t recognized as a virus because it had reached a state of stable symbiosis with the host.
For some months now, there have been loud debates on many media channels about the cultural consequences of transformer-based text-to-picture machines.
AI models such as DALL-E 2, Imagen, Midjourney or Stable Diffusion transfer prompts into arrangements of pixels. They thus generate images that can look like photographs, like drawings or paintings.
*
*
*
*
*
*
*
*
*
Building on the current successes of these text-to-image generators are just-released models for text-to-video generation. Make-A-Video from Meta AI and Imagen Video, as well as Phenaki from Google Brain, take a prompt and then output a video related to that input.
The approach seems promising when we take a look at the latest submissions of research papers at the leading conferences for ML, e.g. the International Conference on Learning Representations (ICLR). In addition to numerous text-to-video submissions, papers are up for open review on research from text-to-3D models or text-to-audio generation.
Student essays
The following essays by ground zero students Benita Martis and Ting Chun Liu explicitly address possible aesthetic practices and cultural consequences of these text-to-image machines:
Poetic materialization – regarding Midjourney
The Transformer Model has dramatically changed the way we deal with arti cial intelligence. The mechanism based on prompting has led us to learn a particular form of communicating with machines. OpenAI said when they released GPT-3, “Wrong answer is sometimes due to wrong question. Prompt Engineering/Design has also emerged to discuss how to communicate with models based on prompting, a syntax that seems similar to natural language but is different because of its command-like nature, like a blurring between natural language and programming language.
Midjourney is a model based on the Transformer framework, built for generating visuals with prompting text. It is currently running on the platform Discord, by joining the Discord channel allows the user to interact with the bot. Simply type in “/imagine,” with the following text as a prompt, and the server provided by Midjourney will return a generated image of based on the text.
From texts to images, the figuration of poetry
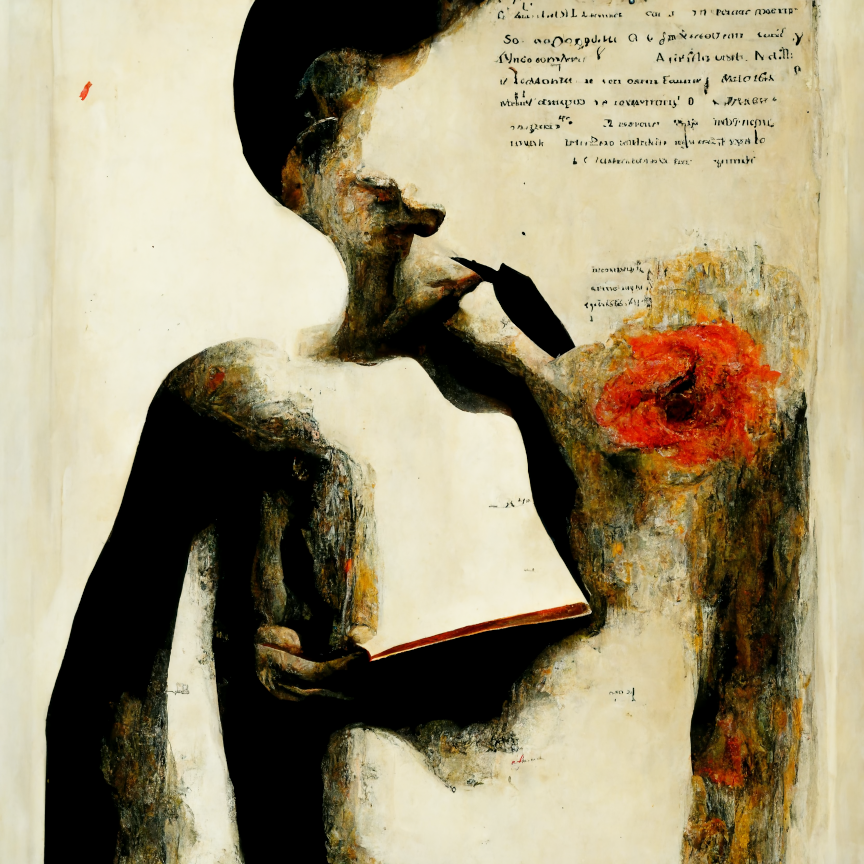
From CLIP to Dall-E, Disco diffusion to Midjourney. Long Short Term Memory (LSTM) was the most common text generation method until machine learning models hit the big time in the last decade. 2019 saw the release of OpenAI’s GPT-2 (Generative Pre-trained Transformer 2), a large language model (LLM) based on the Transformer framework that has shattered the previous imagination of computerized text generation and triggered an arms race for LMMs.
Unlike previous deep learning models, GPT does not have any pre-defined purpose. The basic concept of the Transformer framework is to make predictions by typing a text and predicting the possibilities of the subsequent text to appear. Thus the magical zero-shot prompting became possible: typing a text as a command, GPT automatically produces the desired result without entering any examples.
With the GPT-2 (and its successor GPT-3) being published, a variety of applications have sprung up: the automated writing software OthersideAI, the chatbot Replika, the textadventure game AI Dungeon, and, as a bridge between text and image, CLIP (Contrastive Language-Image Pre-training), also published by OpenAI. CLIP brings grammar in language into image classi cation by using natural language processing as the basis for training. Image recognition is no longer limited to the one-sided interpretation of nouns in images, but verbs, emotion, and tone (although still imperfect) are all possibilities for text output, and the computer thus “understands” language in its way. Although this technology is not the first of its kind, the GPT’s vast linguistic database is a quantum leap to qualitative change.
When image recognition outputs sentences, can text generate images? OpenAI has released Dall-E in January 2021 (since september 2022 available without waitlist), which further lowers the threshold for AI image production. The simple phrase “a picture of a restaurant signboard with curry” will generate the corresponding image. The next arms race thus began, Disco diffusion, Google’s Imagen, and Meta’s OPT were released one after another, all based on GPT-2 and GPT-3. While the next version of DALL-E, namely Dall-E 2 was still in closed beta testing with a few hundred people in Summer 2022, Midjourney came out of nowhere with its gorgeous images, as well as Stable Diffusion, an open source text2image generator which creates images that look like photographs or hand-drawn illustrations.
When linear textual narratives become two-dimensional images, the imaginary gaps between the lines are given space for expression in the images. Dall-E is based on two components, a discrete Encoder that learns how to represent images in a limited space and a Transformer that establishes the parameters of correlation between text and images in the training data. The image and the text are both in the form of numbers in the model, thus making it possible to establish the correlation between them. The production process of the image becomes a figurative interpretation of the poetic meaning of the text. MJ continues to develop this by using a large number of digital art-related creations on the Internet as the basis for training and calculating images.
Replacing labor with heat – Replication, adaptation, and regeneration of image
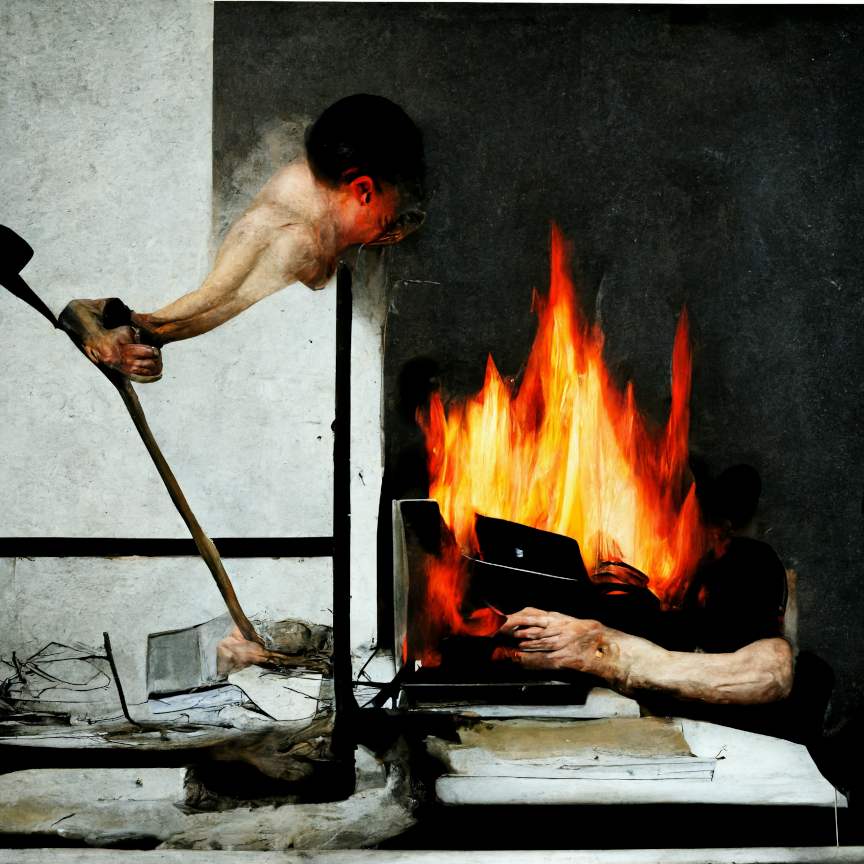
A few weeks ago, while preparing for seminars, I used a computer with a 2080 graphics card to compute a DALL-E image, and with the sound of the computer fan rumbling, six unsatisfied blurred images were produced ten minutes later. The low resolution and the watermark on the surface, which may have been inherited from the training data, are not comparable to Midjounrey’s delicate and complex images. The Discord group generates an image every 30 seconds, and hundreds of users continue to produce different visuals every second. One can’t help but think about the heat production involved. Heat is the main connection between the virtual world and reality. Computers need electricity for all their operations, and the use of electricity undoubtedly emits a lot of heat. Hundreds of complex images are produced, scaled up, and modified in a discord group. Imagine how much computing energy is needed to support this process? And how much heat will be generated during the process?
The formerly exquisite images have become a drop in the ocean of hundreds of generated images per second, and the interpretation of images has been flattend by visual abuse. The work of art is not a transformation of the invisible, but rather, like the transformation of human labor into heat after the industrial revolution, the artist’s labor is reduced to the input of words, the emission of heat, and finally, production. The modulation of temperature in the parameters shatters the only light of the image, and the image no longer exists only as an image but with a pre-determined narration. The technological revolution initiated by CLIP bridges the computer image and the text, and the change of the text leads to a change in the image. The keyboard replaces the brush, the algorithm replaces color, style, and composition, and the same text can be produced in infinite variations.
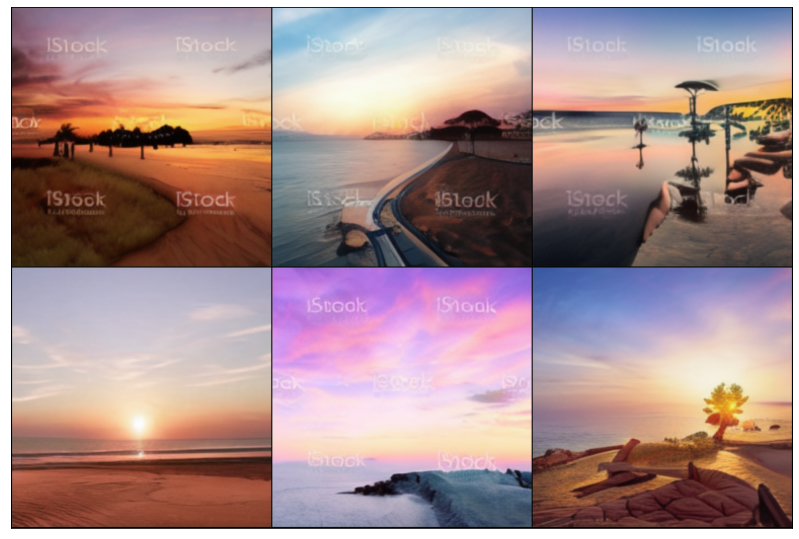
Artists’ last frontier?
I got a Google Sheet a while ago, which shows the Taiwanese analysis of the word frequency of Midjourney users and the possible results of different parameters. From this, we can also see the limitations of Midjourney, which is still based on the imagination of some human database production, and they cannot replace the function of art in the short term. Just like inputting “programmer” will output male, inputting “flight attendant” will output female, and even inputting “slave” will produce portraits that are all black. The new text-davinci-002 engine, updated for GPT-3 in 2021, adds a warning when the output is “sensitive.” All of this tells us that the model still has limitations and that imagination cannot be replaced.
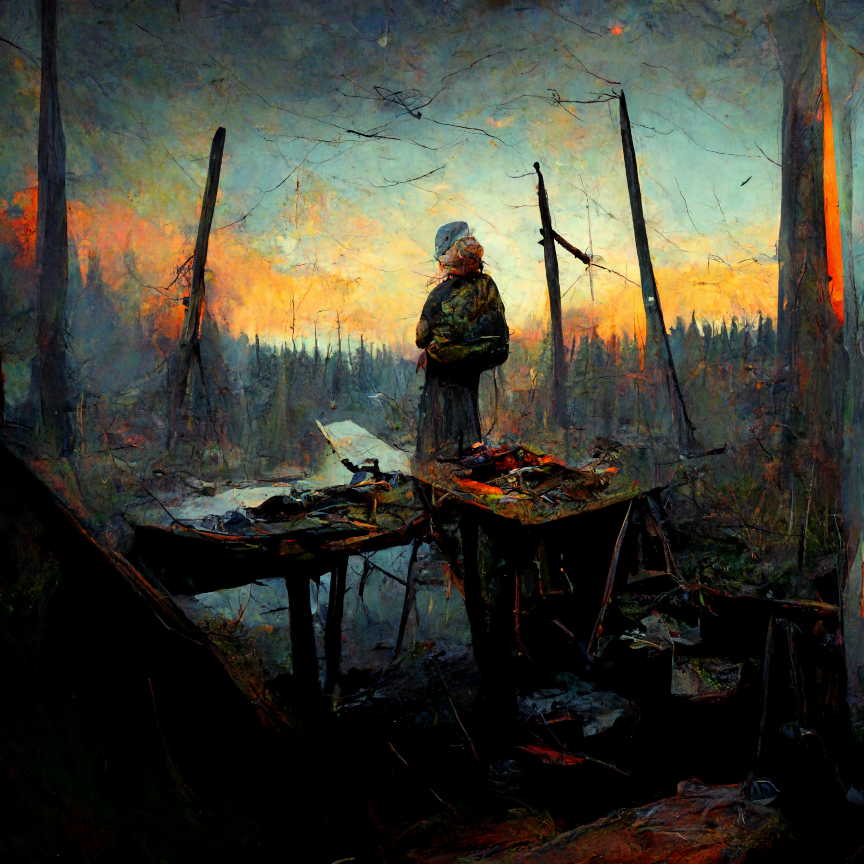
Five years ago, Generative adversarial network (GAN) hits the art market. As the first GAN-generated images were printed and sold in 2018, the irreplaceable defense of the human mind vs. Machine has been shaken. Five years later, artists are still around, and the artist is relieved that NFT, an algorithmic art based on human programming, has become a huge hit, with Midjounrey generating a large number of exquisite images that include many #DigitalArt works from Pinterest in addition to traditional paintings as training material. We can argue that Midjourney is only capable of accomplishing a speci c form of artistic expression. This eye- catching effect may seem to destroy the artist’s work, but in a way, it frees up the labor of image generation and even extends the method of labor. GPT-2 has been around for nearly three years and has not eliminated the writer’s presence but rather has become an aid to writing. These devolved technologies can be imagined through Bruno Latour’s Actor-Network Theory (ANT), when the language-graphic generation model becomes a new aid for the creator, and the creator, text, and artificial intelligence model construct a new actor-network for image production. Remember conceptual art in the history of art in the 1960s and the use of text as a carrier of concepts to produce “images” of art. When the viewer reads the images and documents of conceptual art, the logical thinking in his or her head is the expression of his or her artistic concept. For example, when viewing the images produced by Midjourney, one cannot help but think about what words were entered during the initial prompting. What kind of changes can be made to produce the images?
Currently, regardless of Disco Diffusion, Dalle-E 2, Midjourney and Stable Diffusion, a large number of generated images have some degree of similarity, and their own artistic style (or Bias so to say). It rised up couple questions: When these images are returned to the web through #AIArt, what kind of landscape will these training data generate again? Since the work of text is also the work of an image, the artist’s job becomes to discover and explore the connection between these materials. When every single second a new complex graphics are produced, the value of art will be refined in the process. Can images be more than visual but as carriers of concept? These questions will be valuable only after the Machine replaces the labor of art.
INFORMATION WAR – War perception and propaganda in social networks using the example of TikTok
The media production of war images may determine the users individual attitudes and behavior towards war. Influencers on TikTok thus have an active political impact that goes beyond social media. In addition, the platform plays a significant role in the Ukrainian war due to its high reach. Ukrainian influencers often show very personal and direct insights into the everyday life of this war, while some Russian influencers spread state propaganda. They are Putin’s digital fighters in the information war, while TikTok is a strategic tool for spreading propaganda of autocratic regimes like Russia.
Human or machine – a classic
The recycling of algorithmic interpretations through re-compositions in text and image, shows varying degrees of artificial “intelligence”. Large pre-trained AI textgenerators (LLM), such as GPT-3, are capable of generating propagandistic texts that at first glance can hardly be distinguished from human-written propaganda. Only on closer inspection do syntactic and semantic errors point to their artifocial origin. The training data of an AI are of great importance, as they often show problematic characteristics, such as derogatory references towards marginalized groups, as well as the reinforcement of stereotypes. Di erent social groupings and their behaviors thus produce differentiated learning data for the algorithms, leading to subtle but highly questionable text generation processes. Computer-generated images, on the other hand, contain obvious errors and distortions; their reception is very different from our usual perception, or photographic images. But we are at a turning point. It is already possible to generate deceptively real images and videos with little effort, which favors entirely new and faster ways of spreading propaganda (f.ex. Deepfakes) virally on social networks. AI-supported tools for image and text generation are becoming a powerful political instrument.
The pop culture veil
The images to be seen are stills from TikTok videos under the hashtags related to the war in Ukraine (such as #saveukraine #russia #stopwar) from female influencers. An algorithmic categorization by hashtag, VideoID and SoundID forms the basis for the artwork with the videos. Here, a commercial Image-recognition software from the company clarifai is labeling the Video-Stills afterwards.
Such Start Up Companies selling services with their AI-Apps that have “learned” to describe images in detail. These algorithms are also applied in social networks, for example to filter violent content. However, TikTok videos often obscure what is being viewed due to their pop-cultural aesthetic transmissibility. Not only for human viewing, but also for the AI image recognition techniques just described. Bombed houses in the background of a Ukrainian war-influencer, for example, are not recognized as such.
Using a Russian version of the DALL-E image generator (ruDALL-E) and based on the previously collected algorithmic image descriptions, the “new” artificial female Influencers are created. The model for the Russian AI is DALL-E from OpenAi and Microsoft, like already described above, one of the first AI’s that generates realistic images from short prompts. The differences between Western and Russian algorithms and datasets are deliberately exploited here. Through the associated hashtag of the original video, a direct link is established between social networks and the generated images.
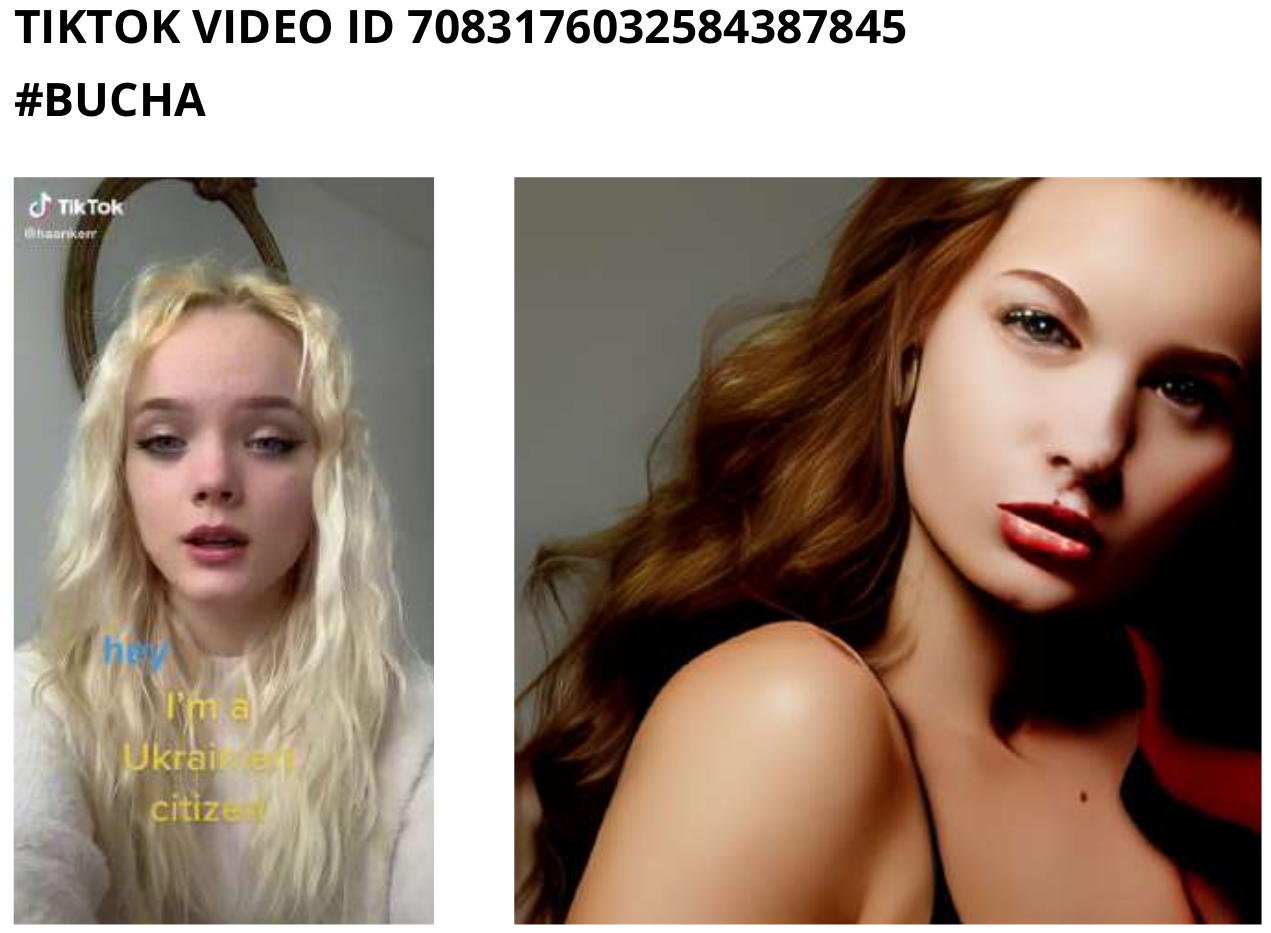
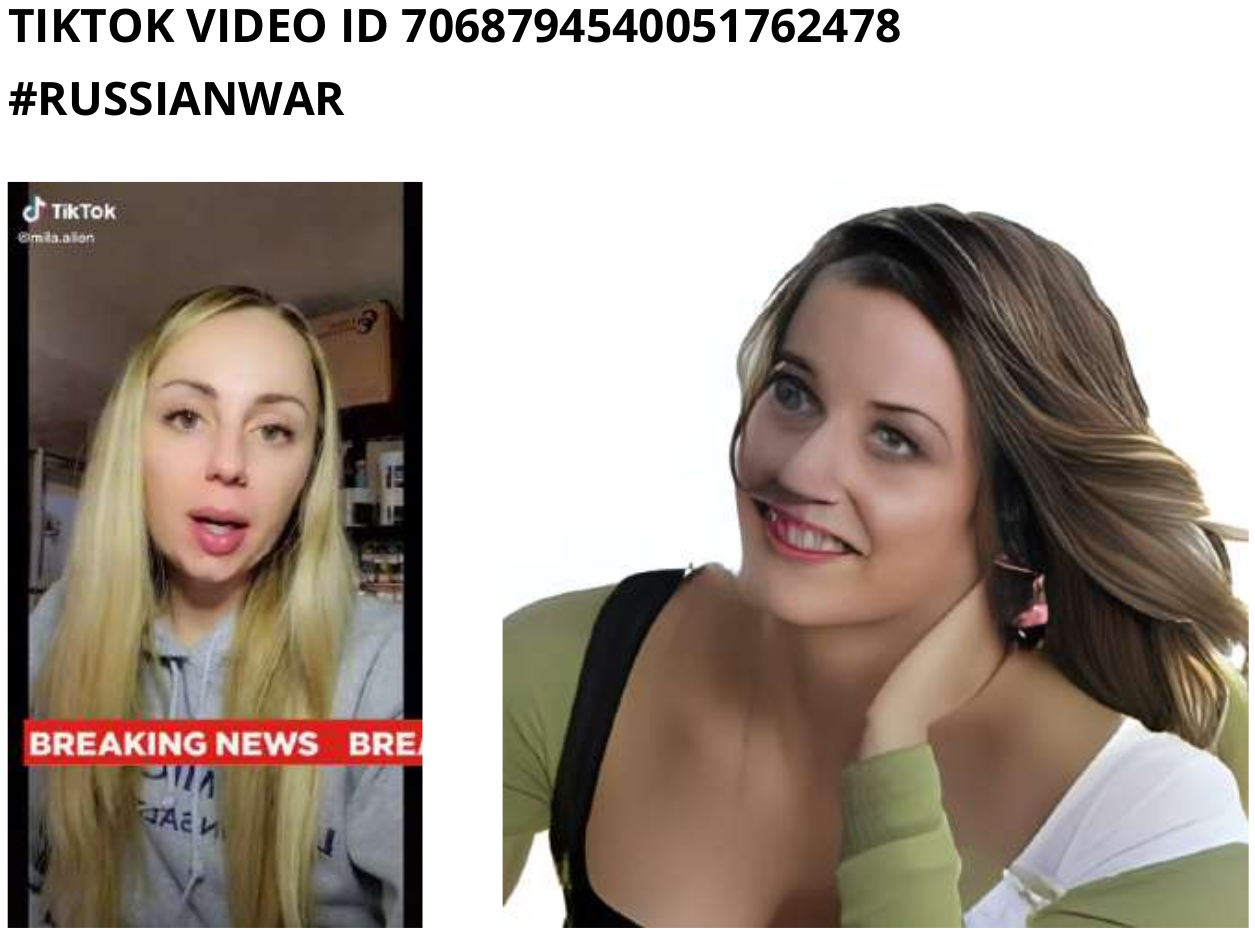
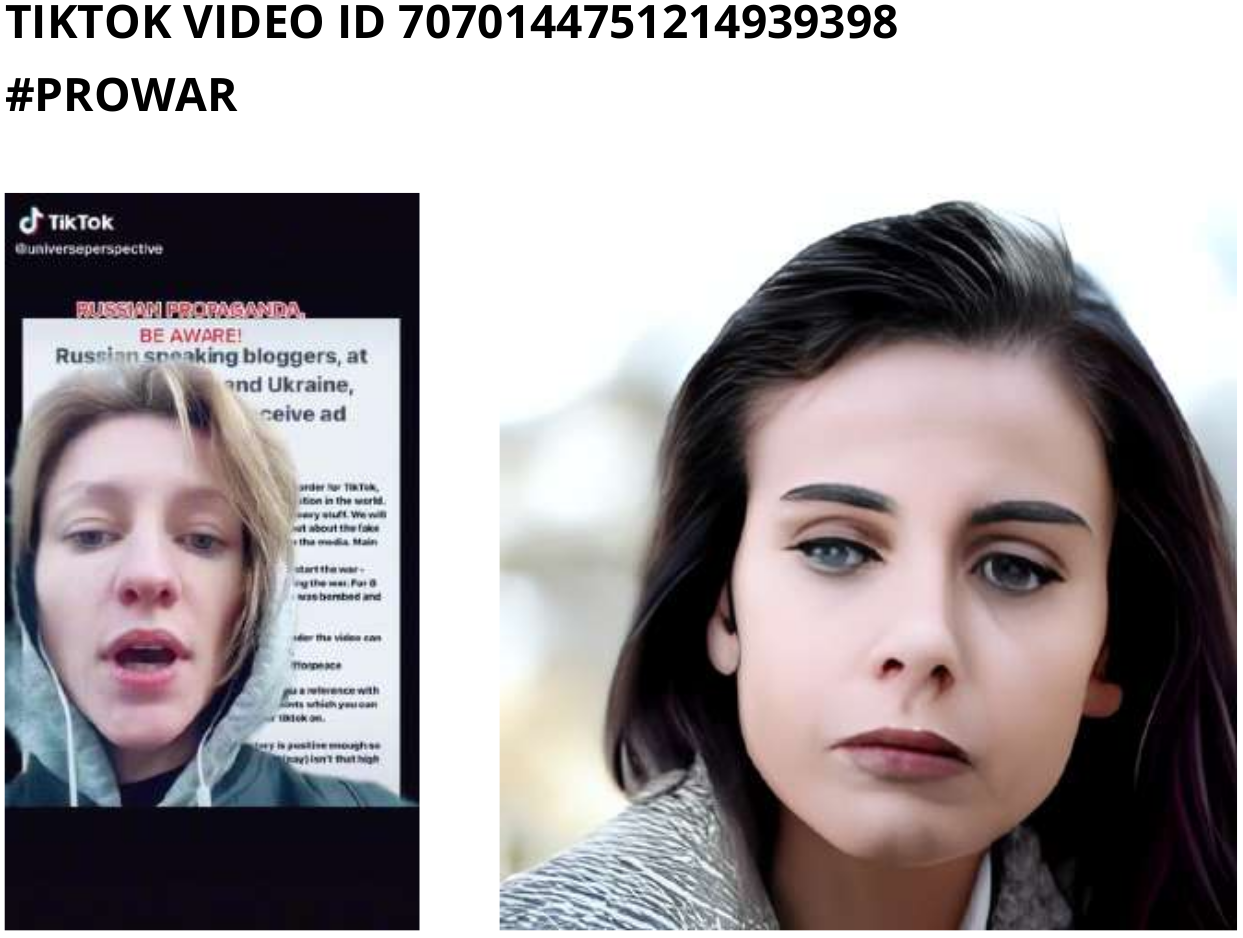
Am I allowed to do that?
In this context, the decisive question arises as to how far art may make use of images of war in order to have an impact on society. The alienation of war images through the pop-cultural aesthetics of influencers is contrasted with the equally alienating aesthetics of AI algorithms. Through the experience of difference with AI-generated images, the project appeals to our self-reflection in order to enable a critical consideration of our interaction with social networks in times of war.
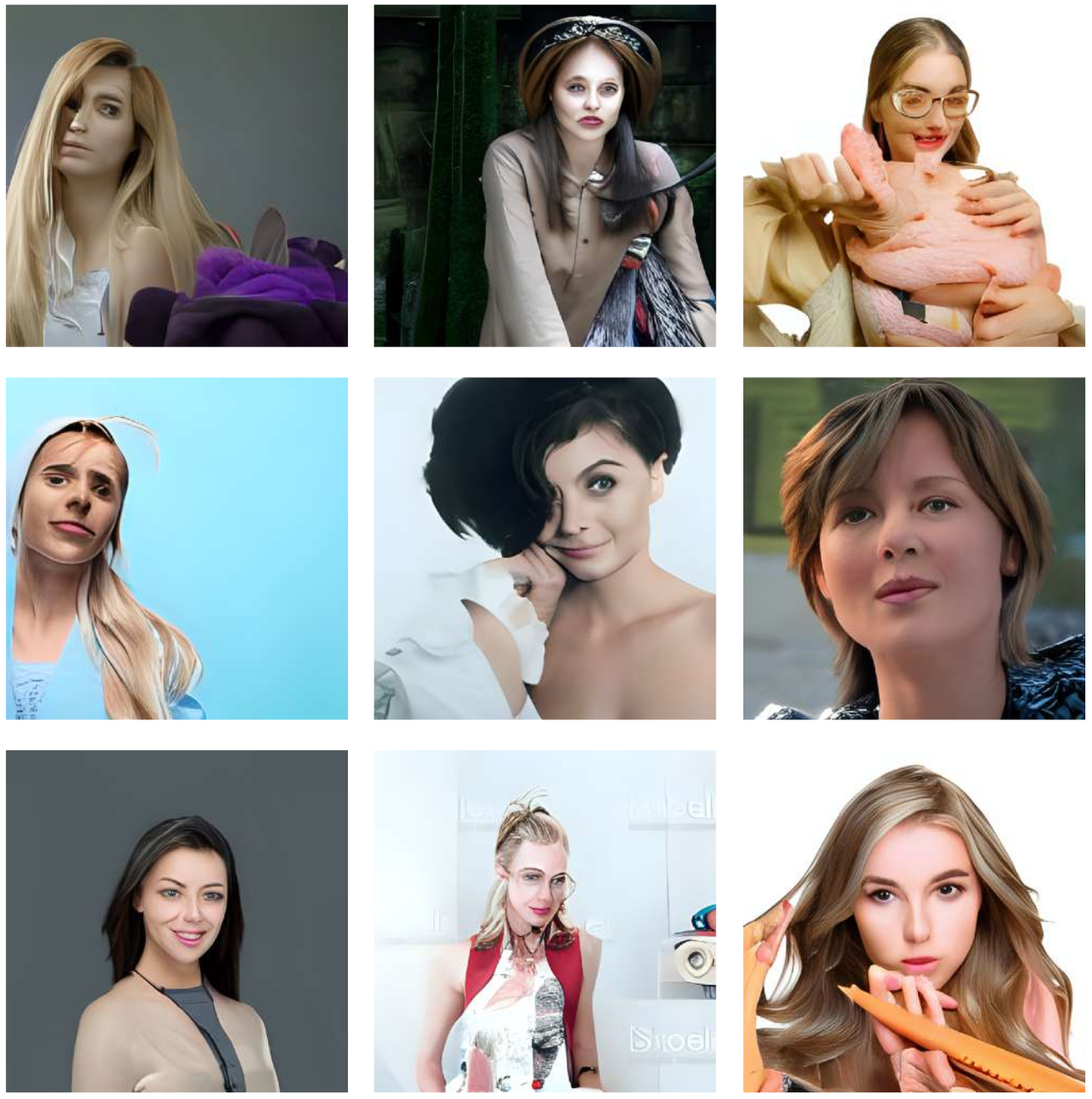
ground zero review WiSe21/22 – SoSe 22
Seminare
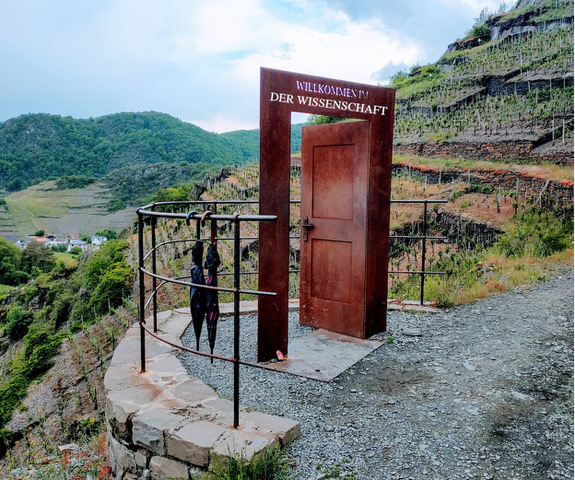
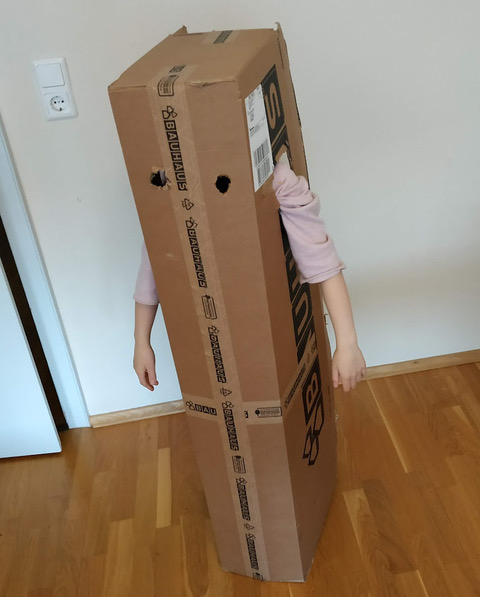
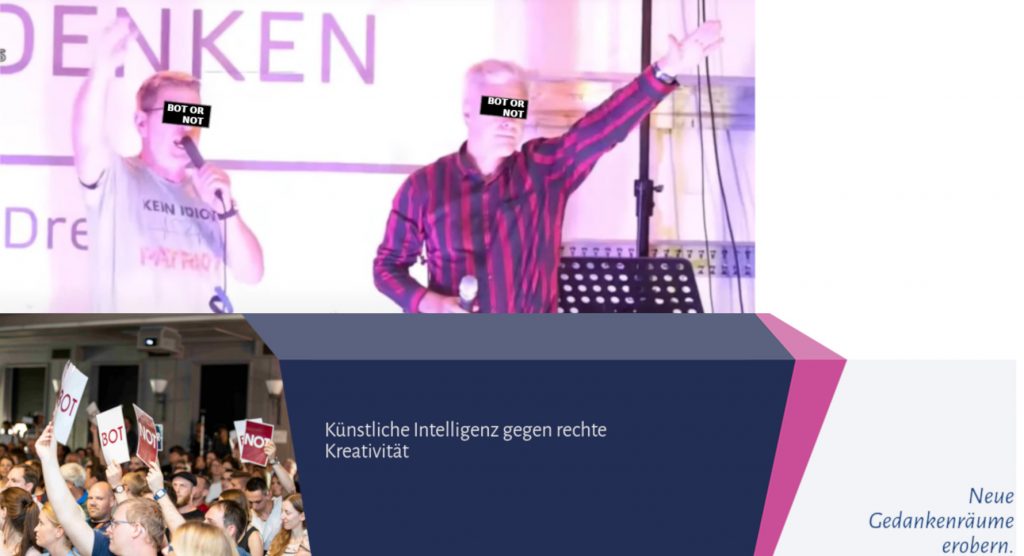
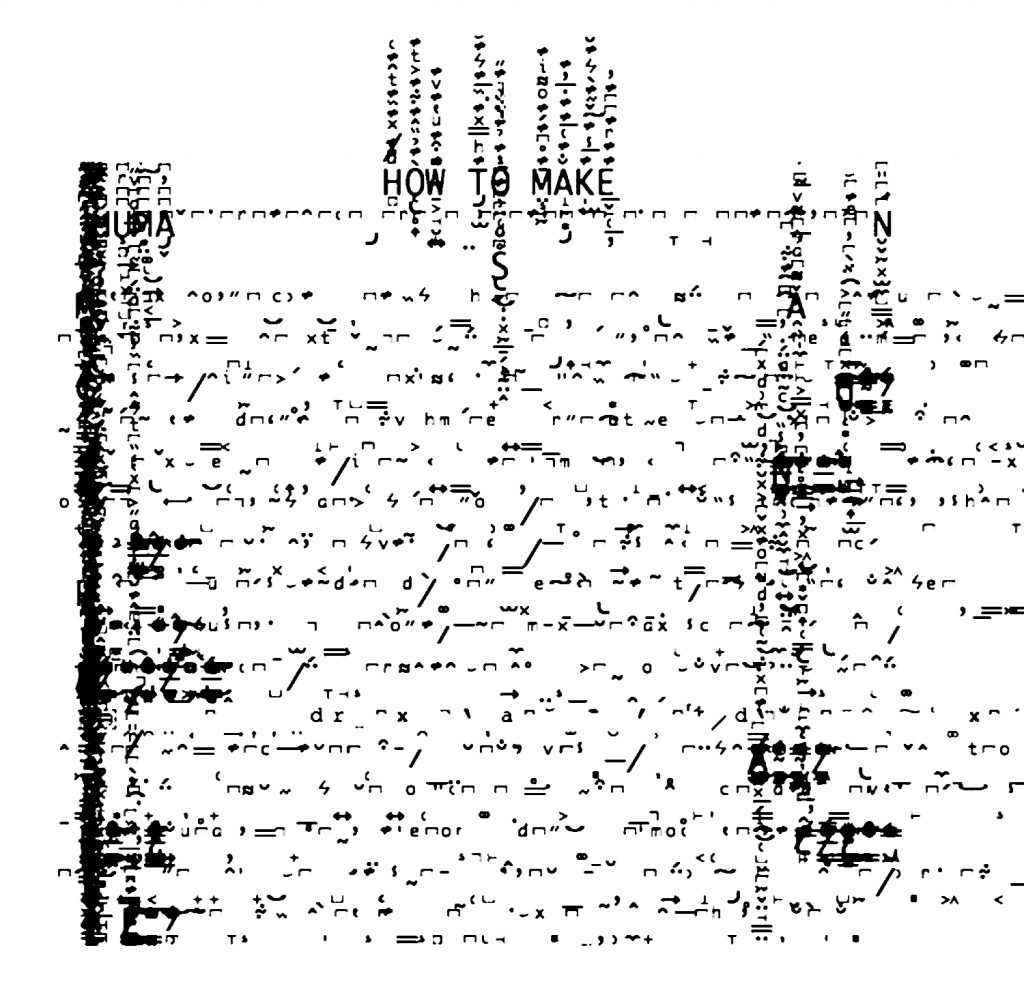
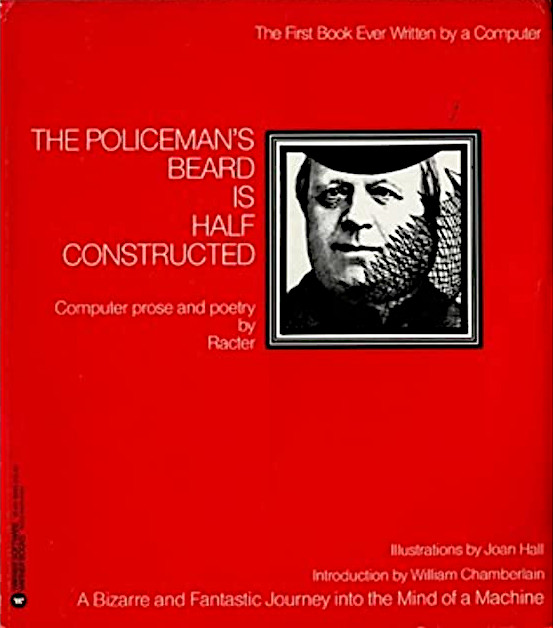
Workshops
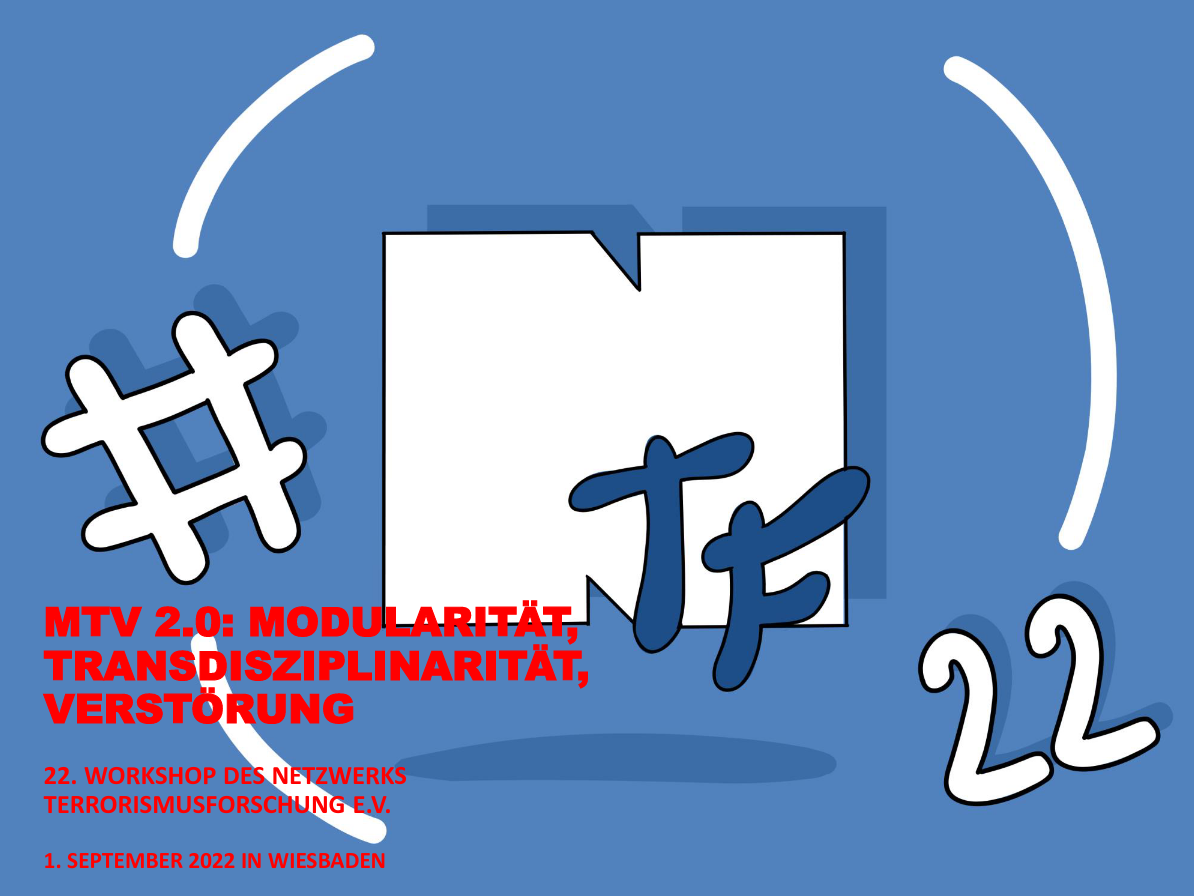
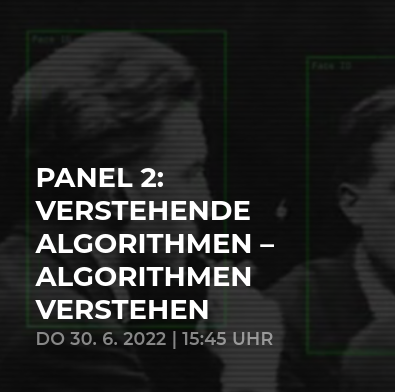
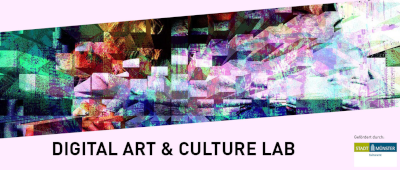
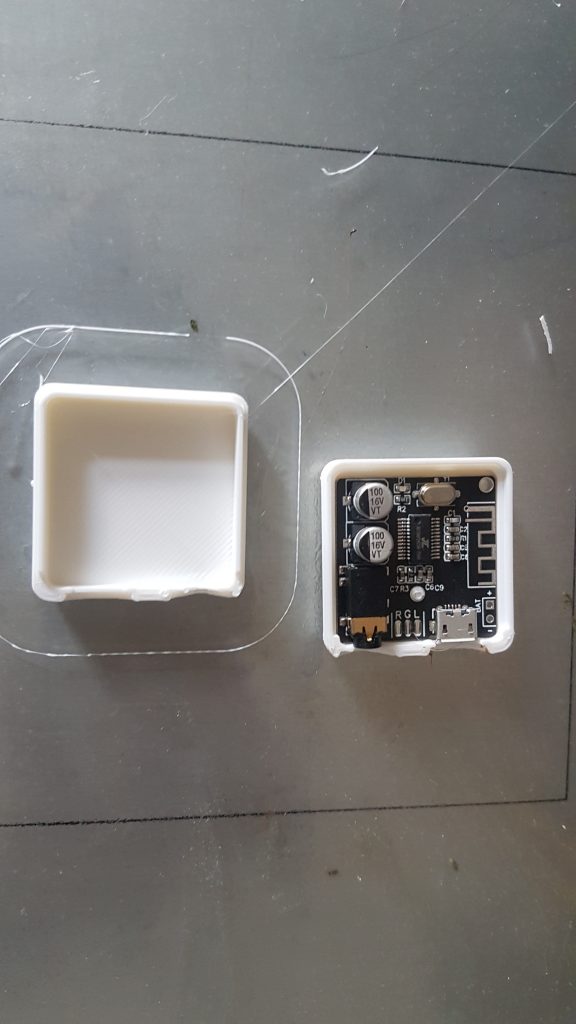
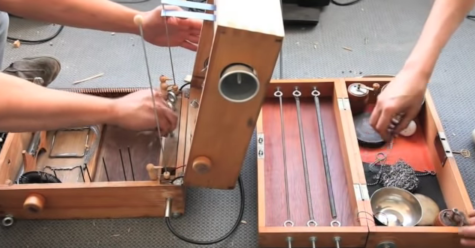
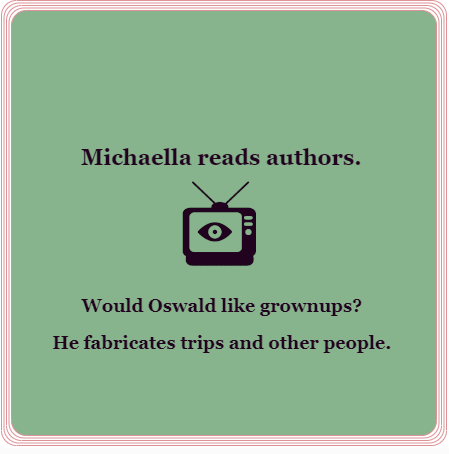
Shows / Exhibitions / Releases / Publications
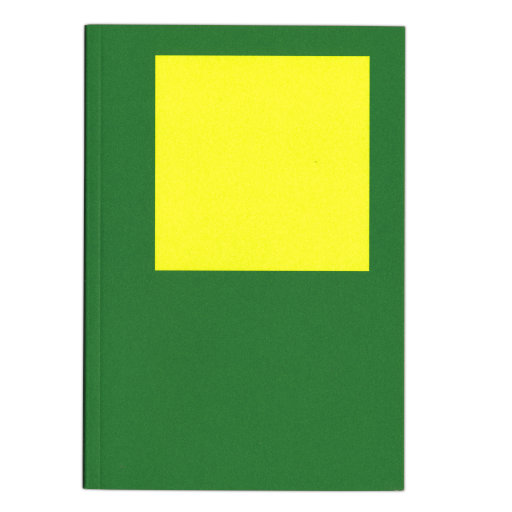
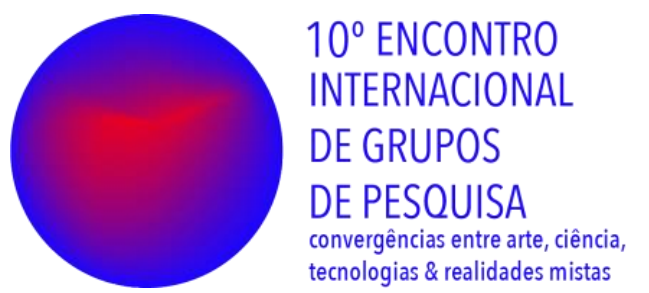
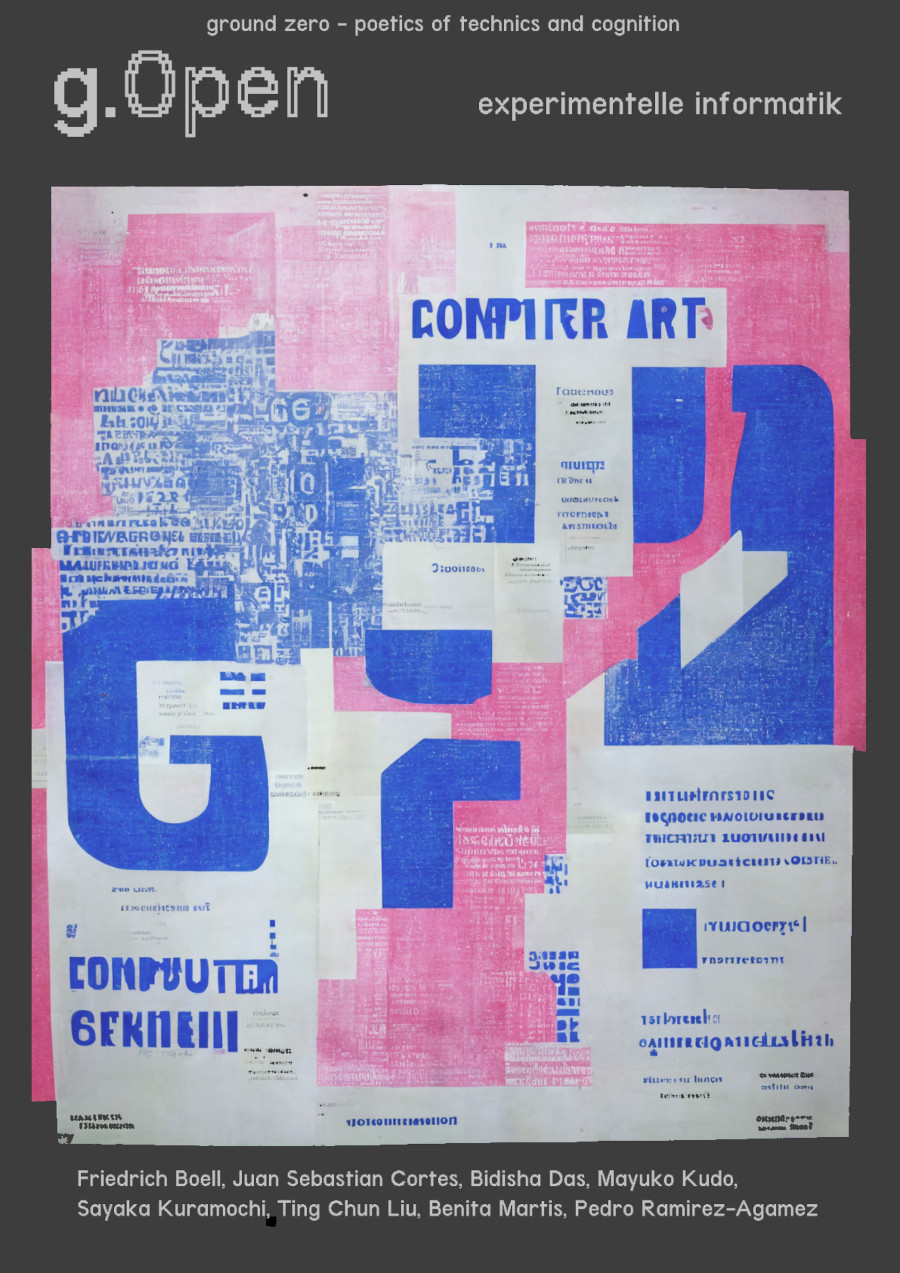
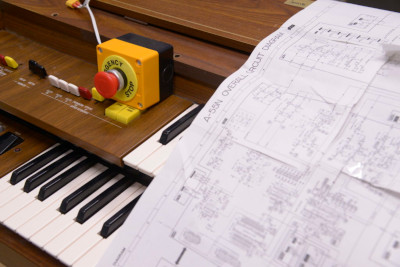
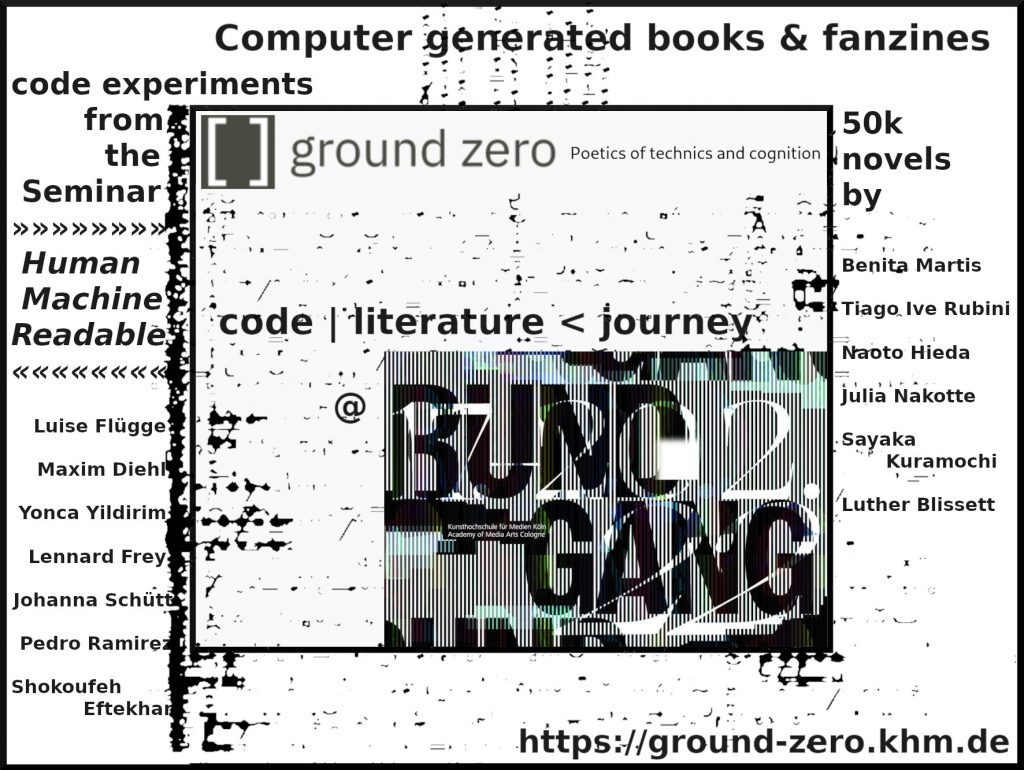
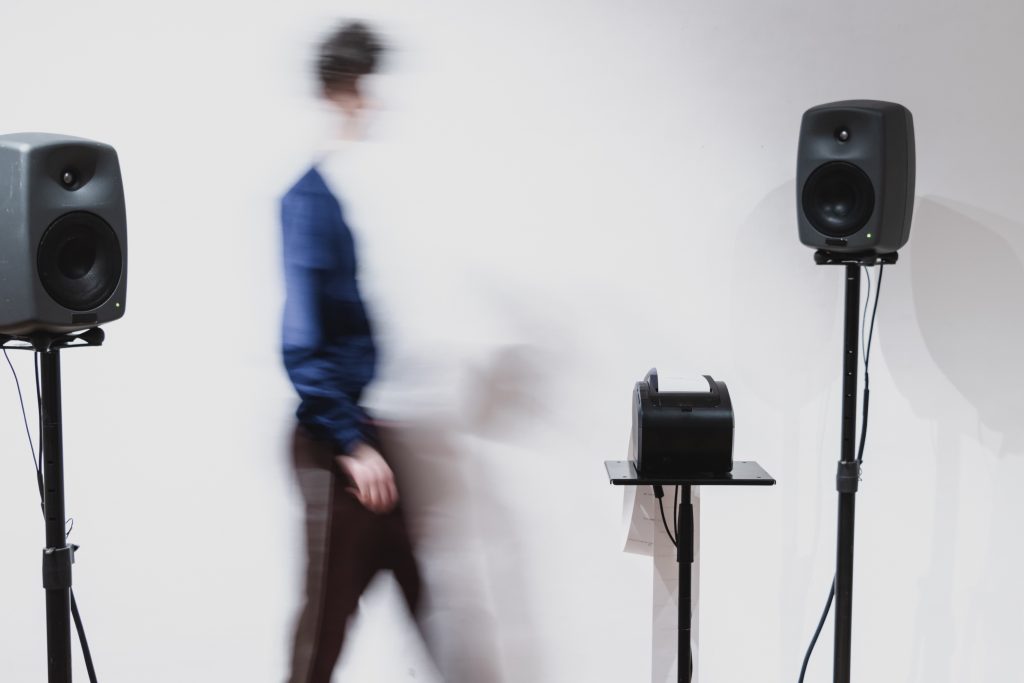
Talks & Conferences
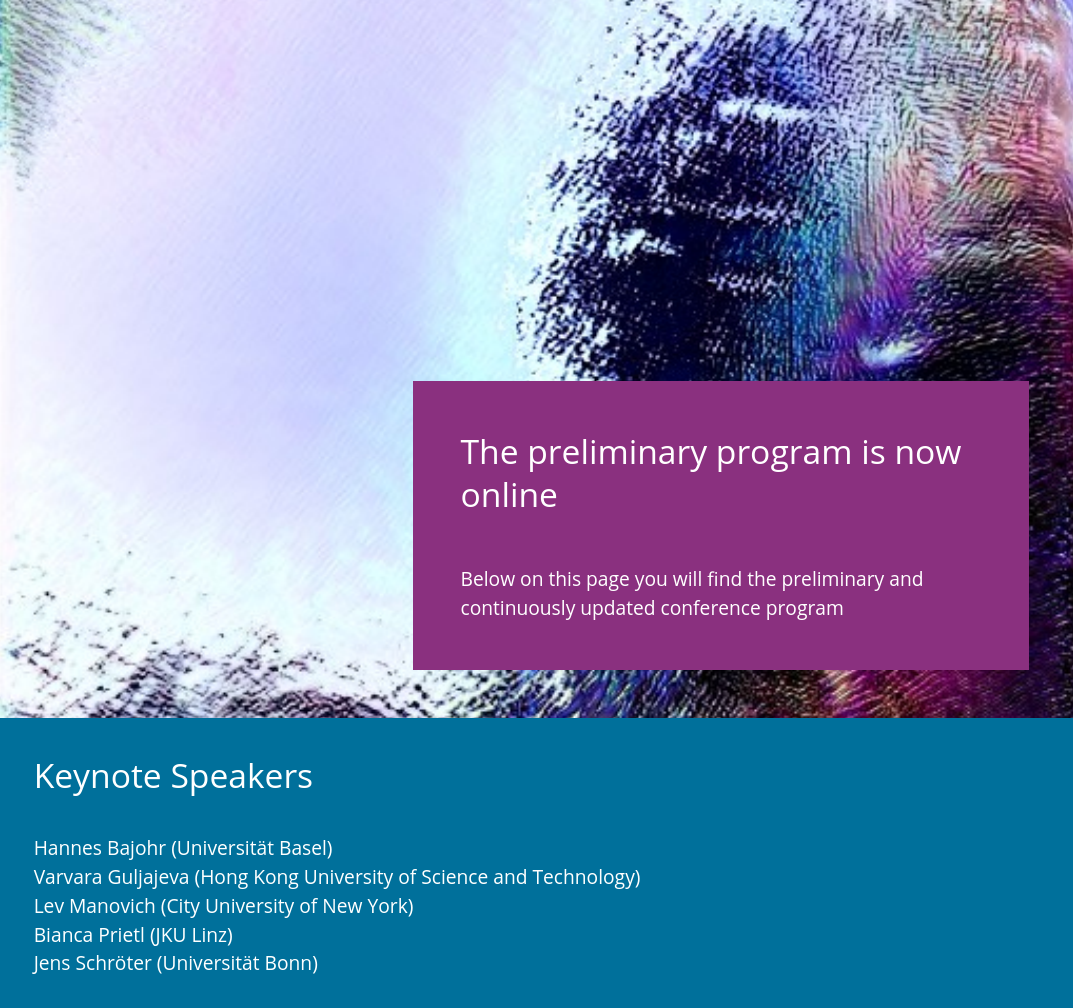
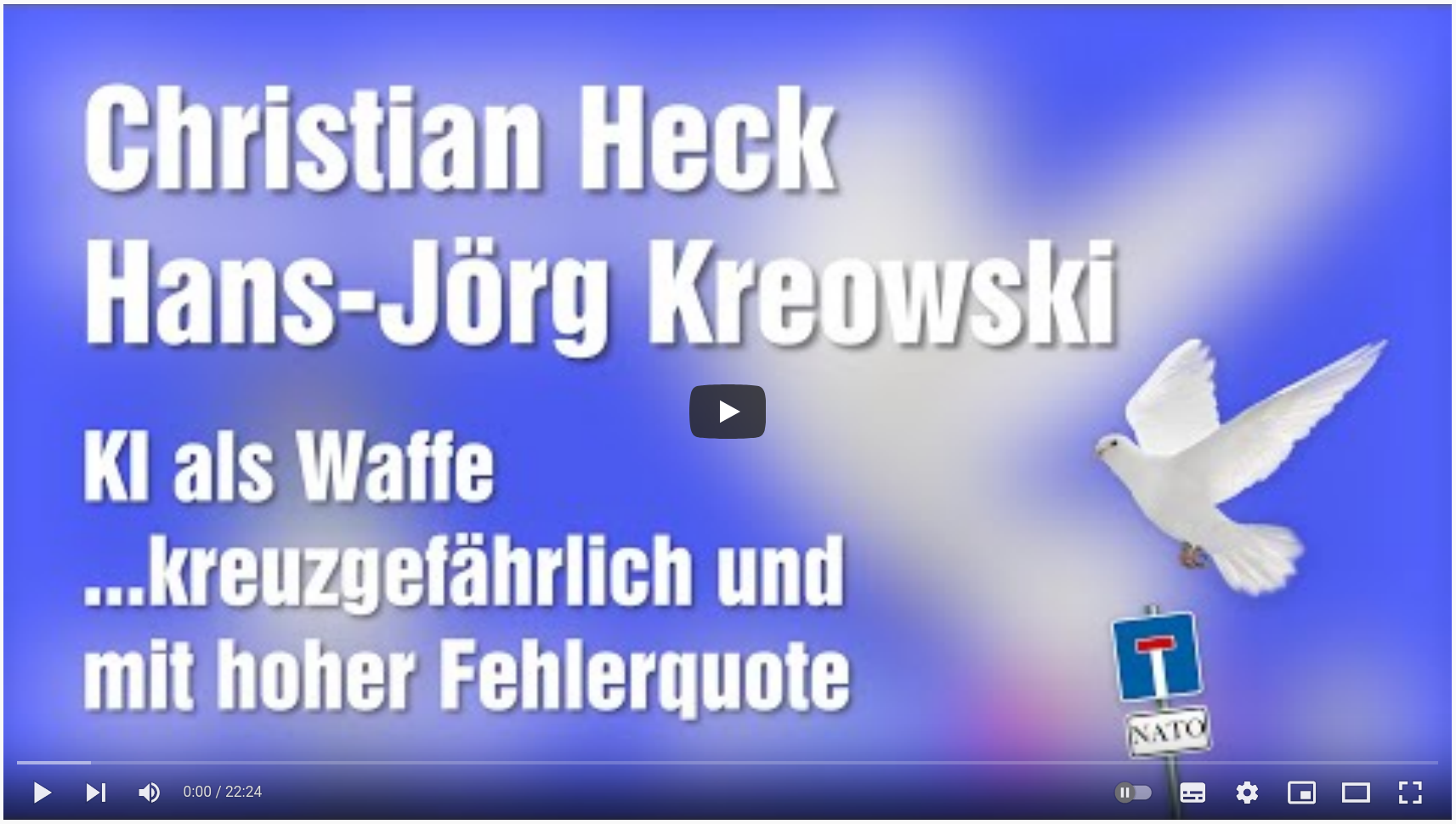
Artificial Intelligence as a weapon (FIfF) @ Living without NATO – ideas for peace
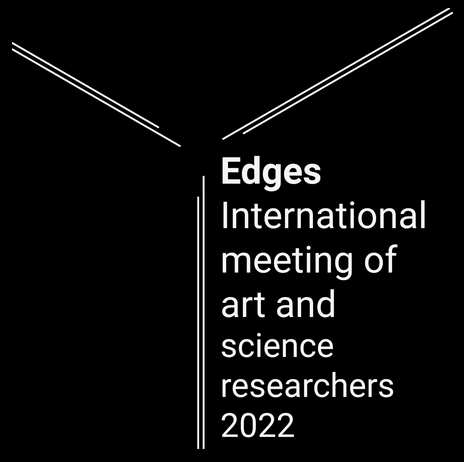
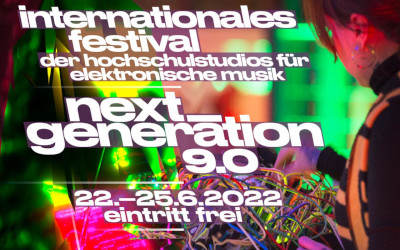
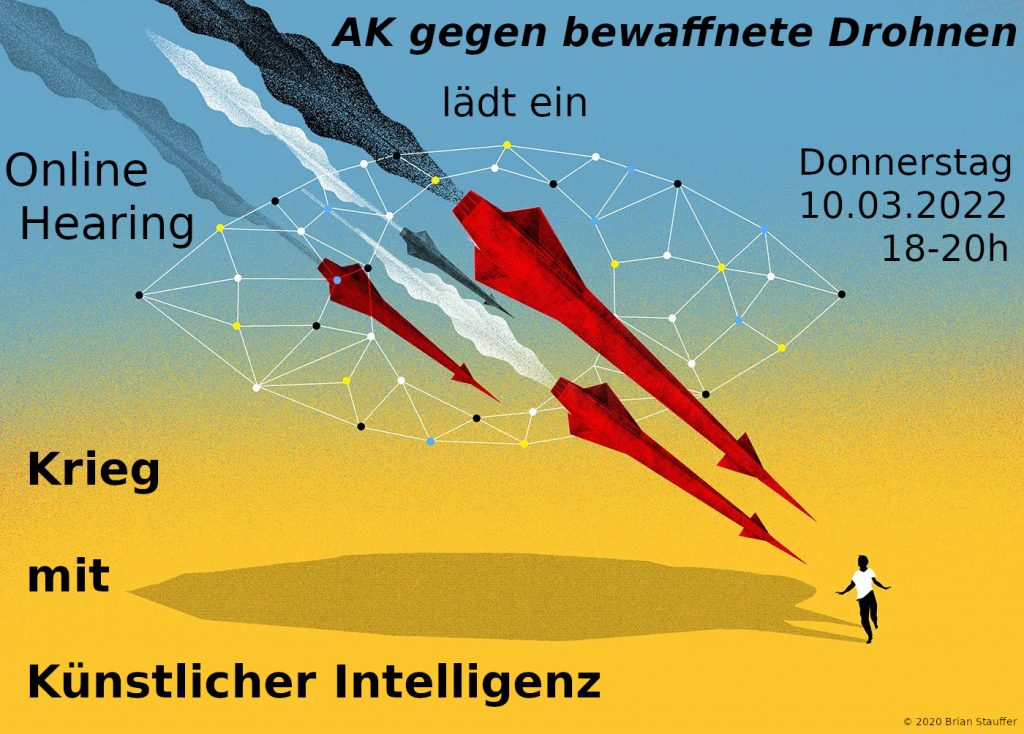
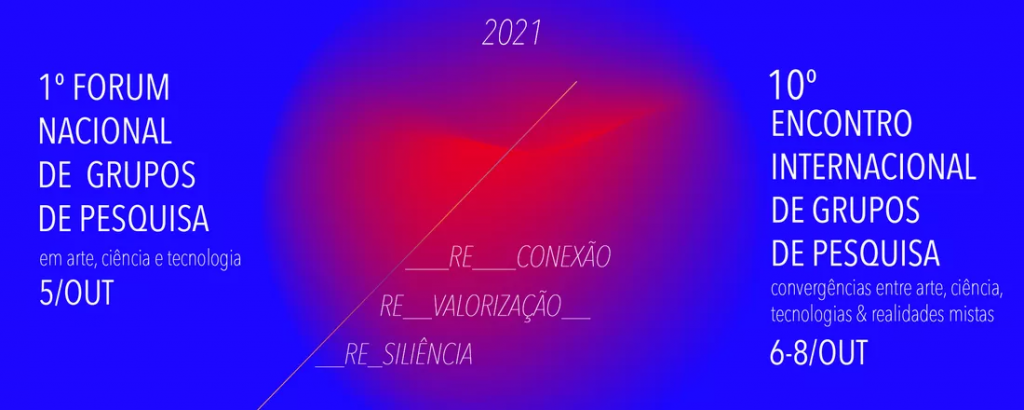
Software Releases
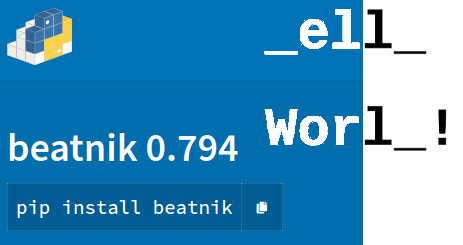
ground zero preview WiSe 22/23
Seminars, publications and Workshops
Seminars
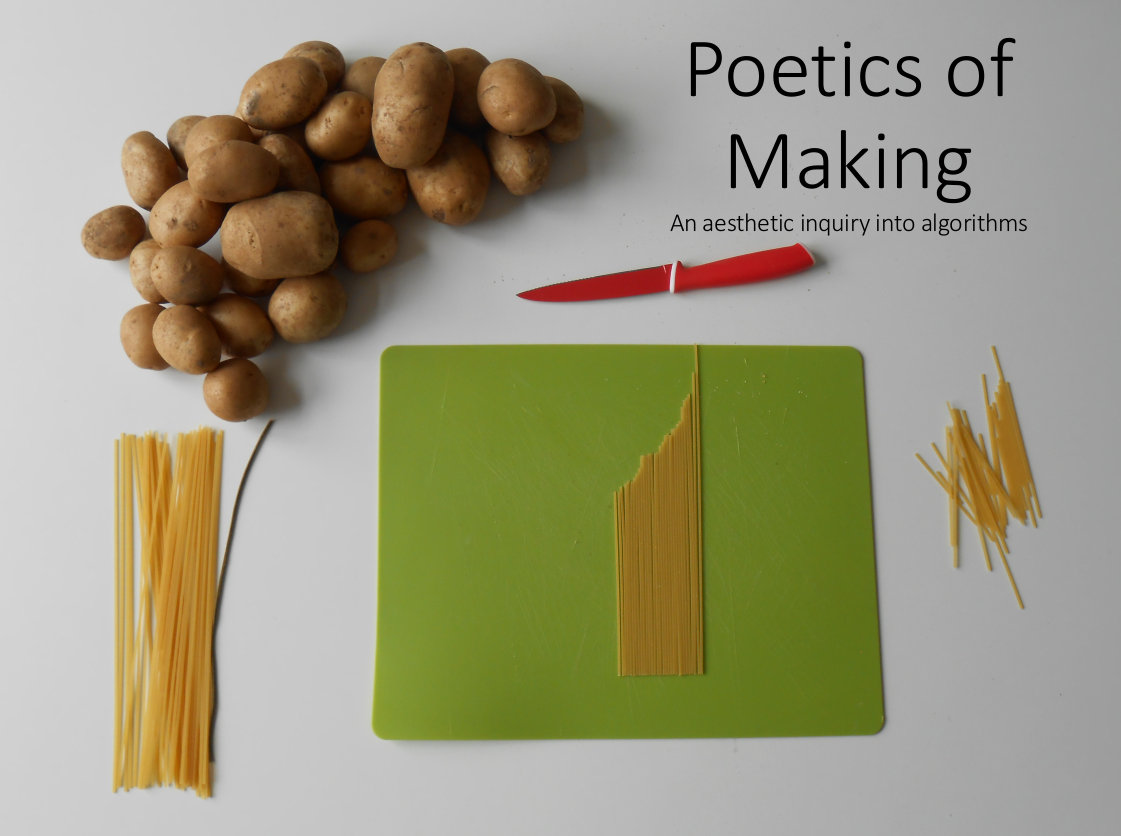
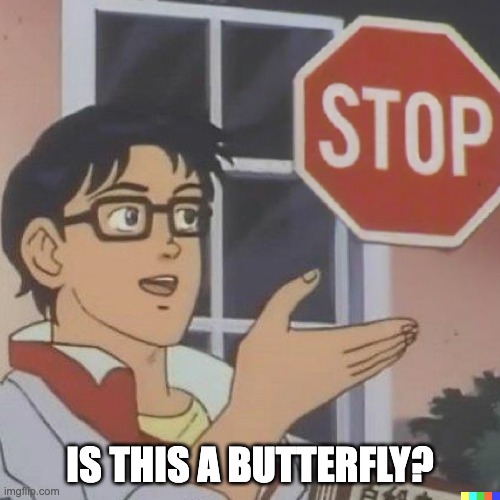
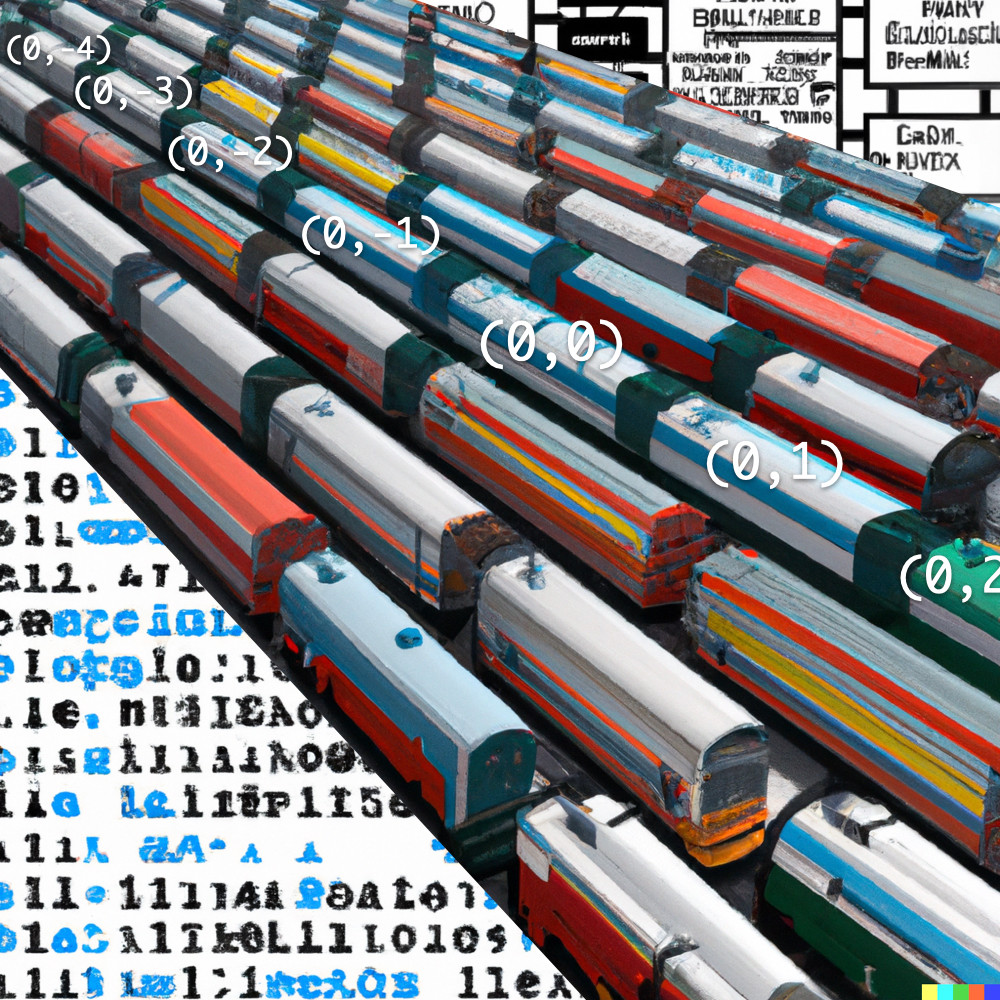
Publications
Workshops
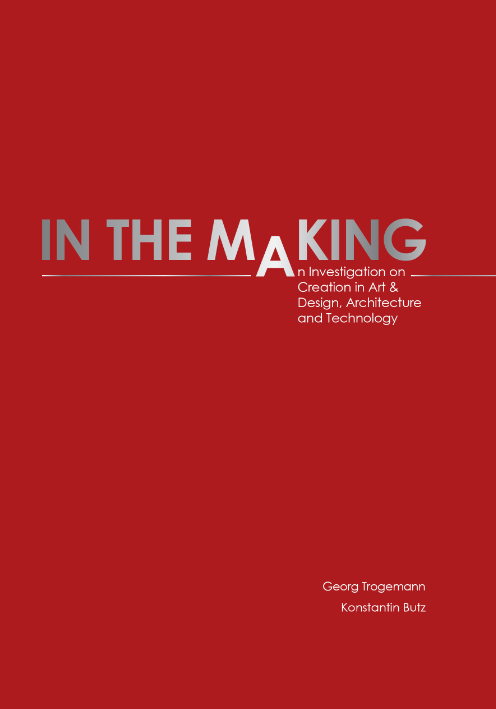
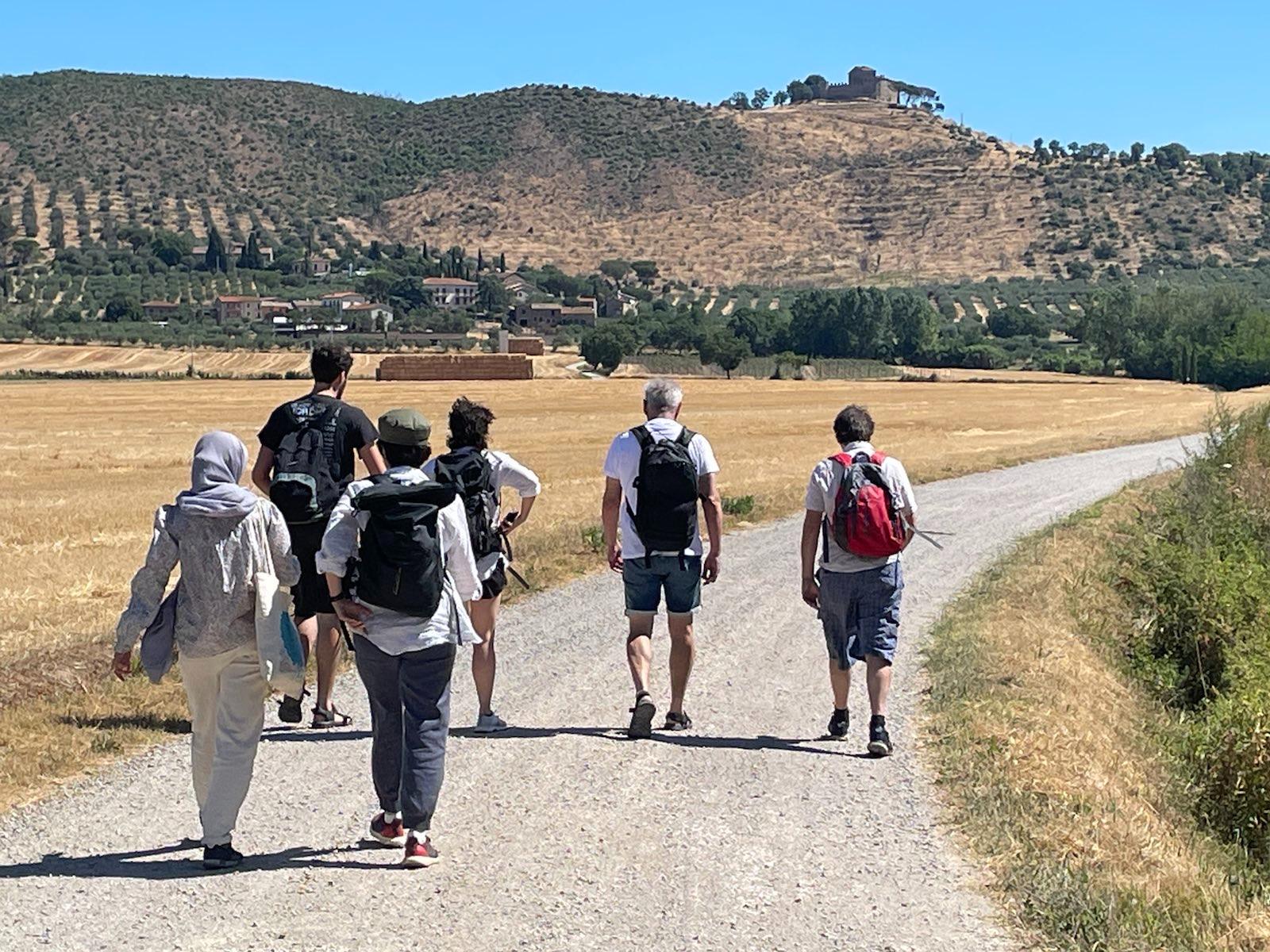
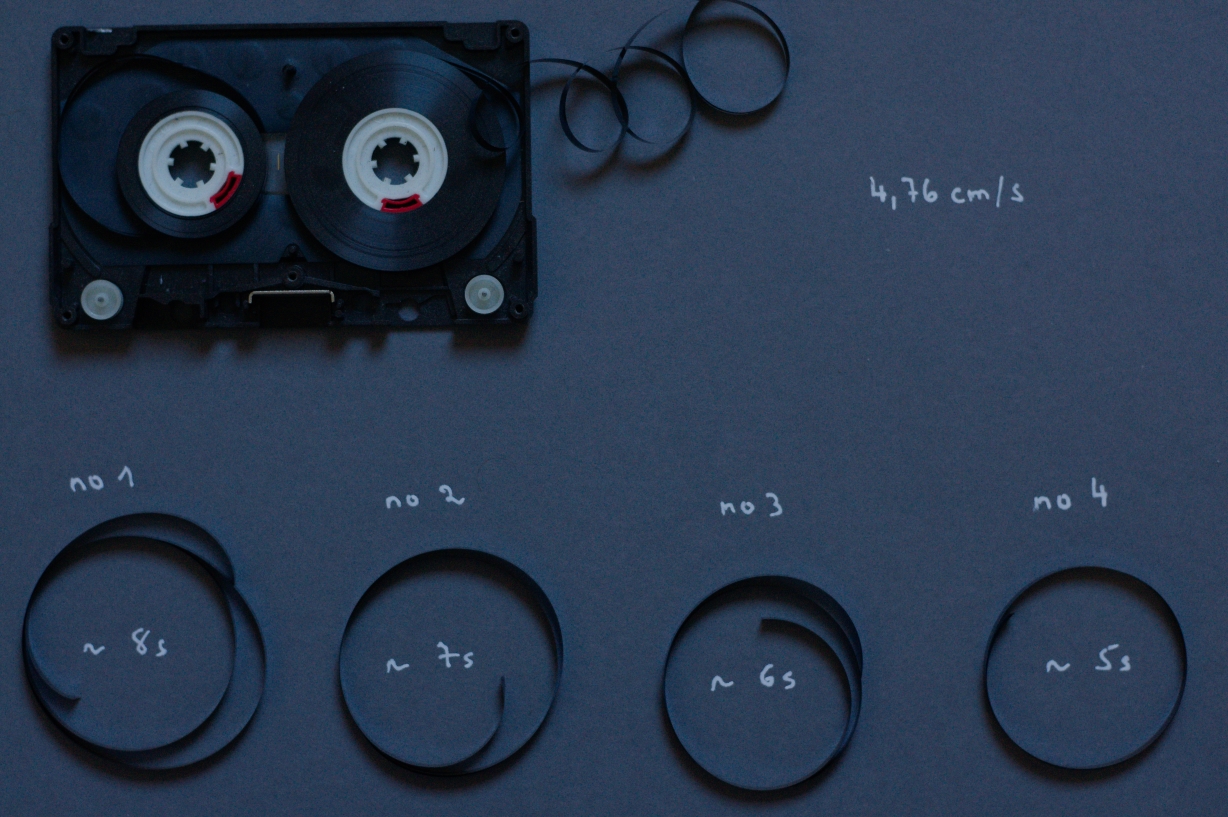
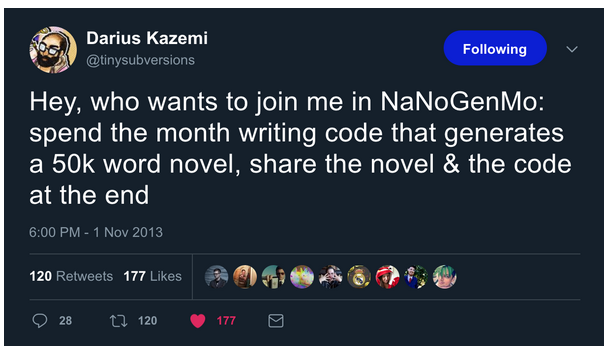
Upcoming events in the closer area of ground zero
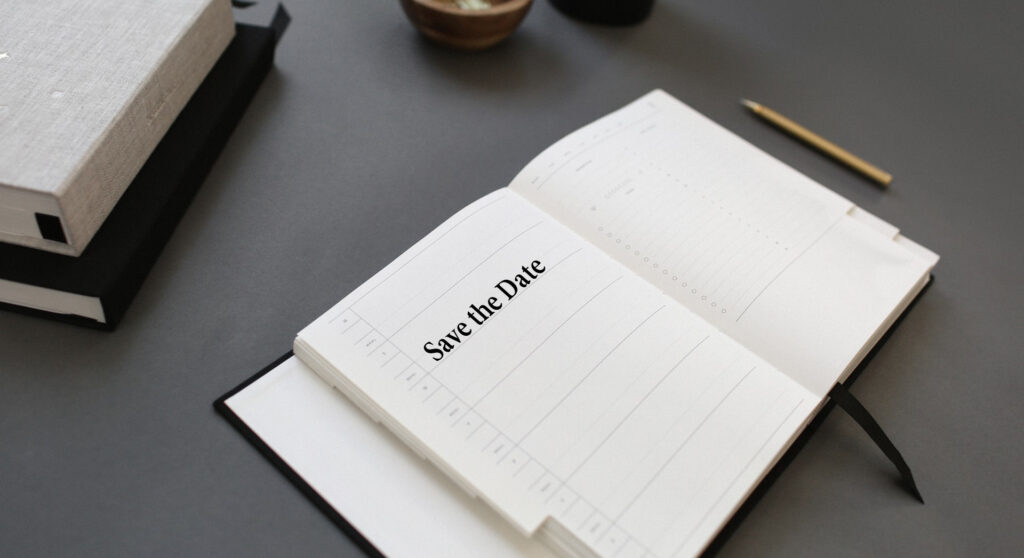
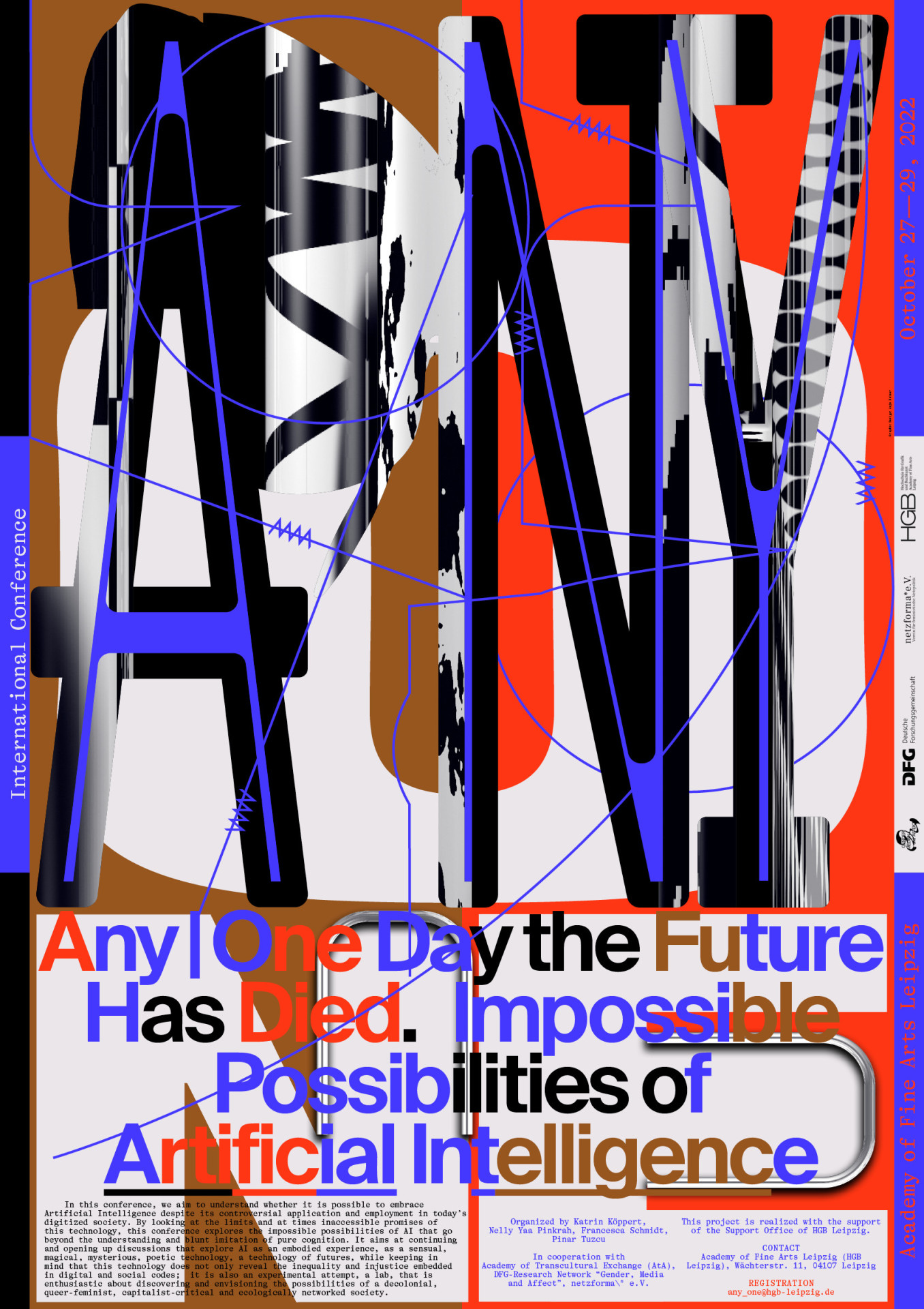
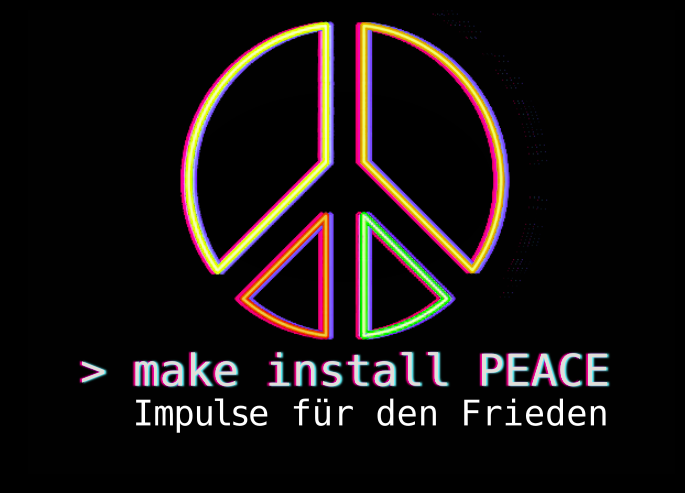
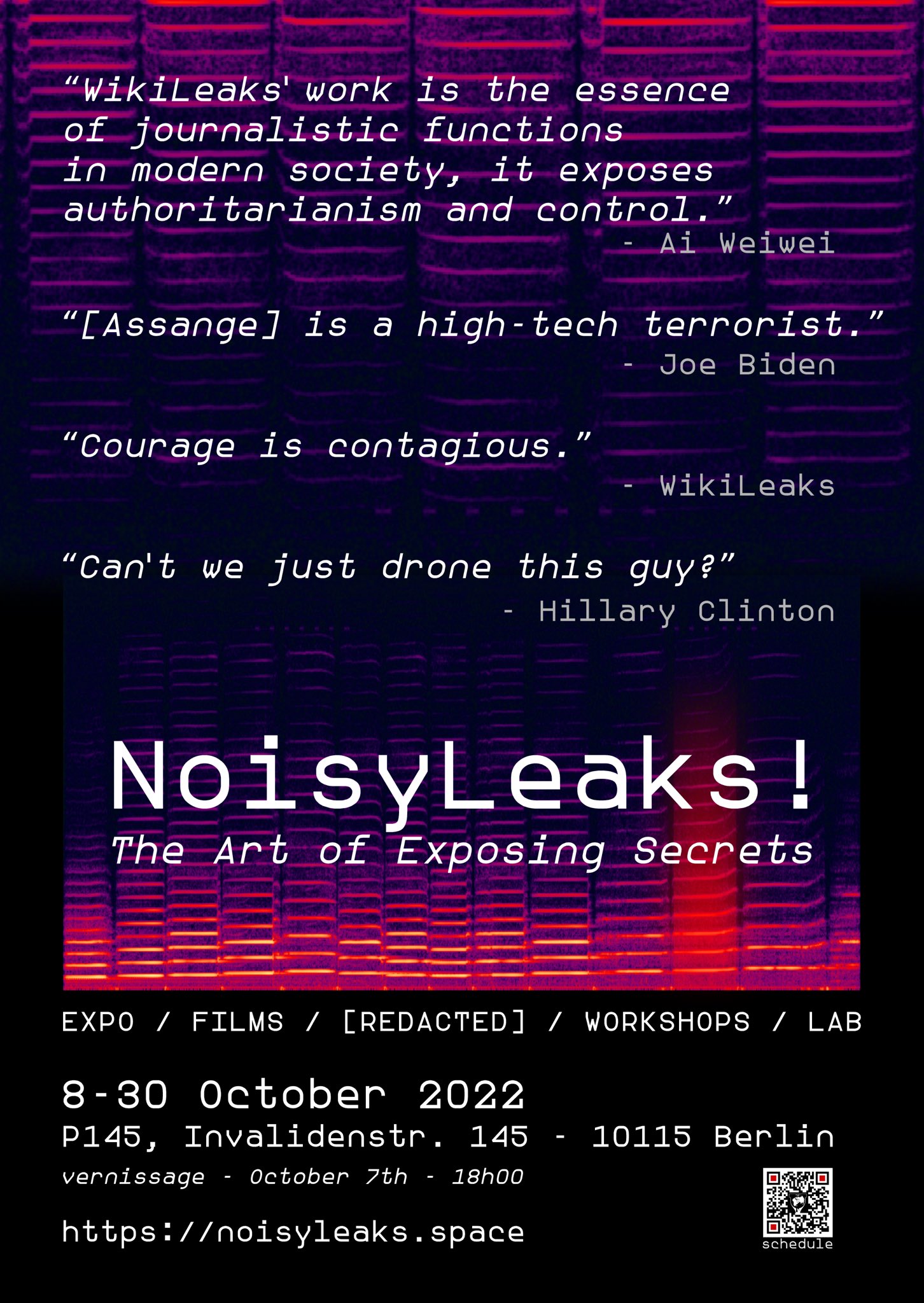
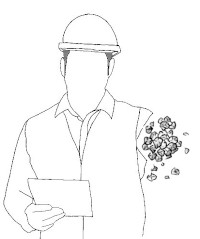
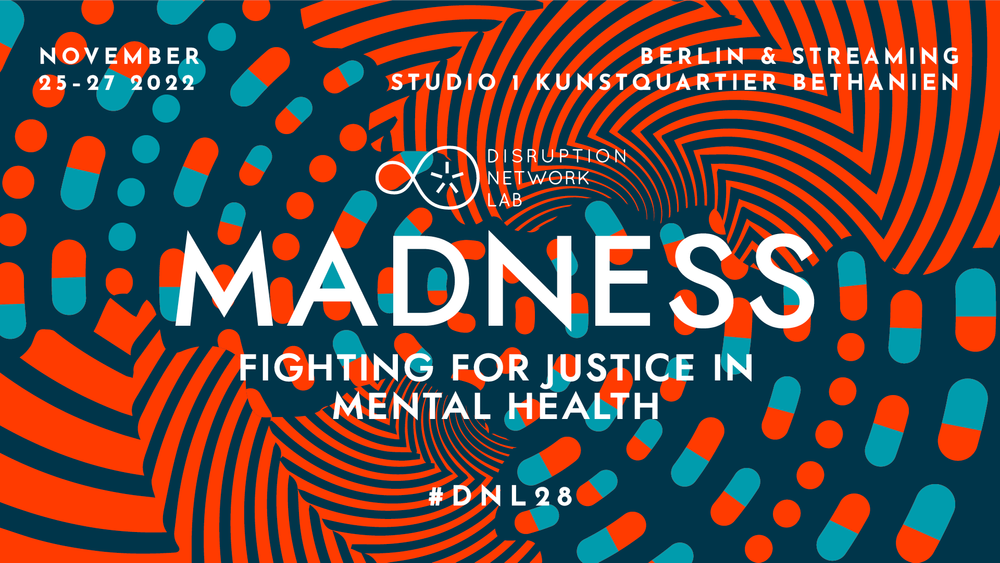
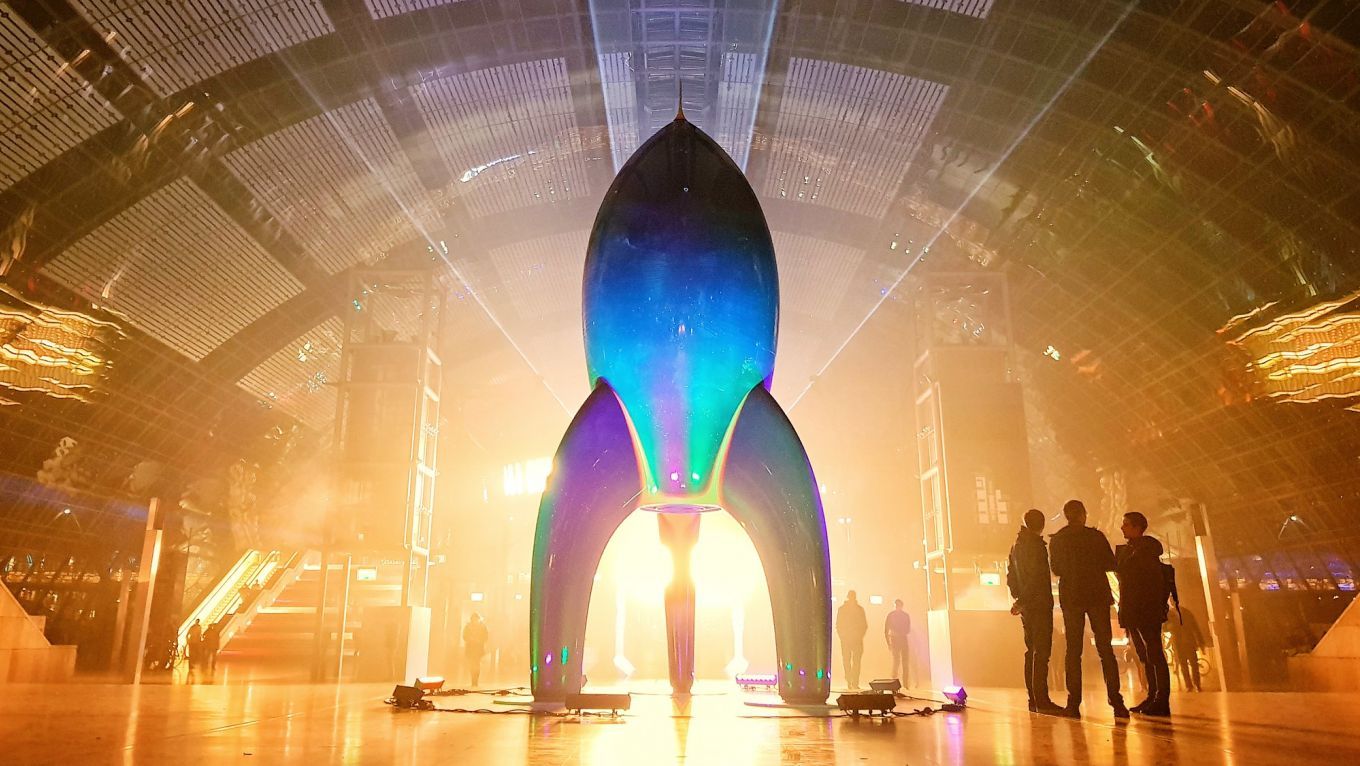